Lecture Series: Medical Information Sciences
Medical Information Sciences
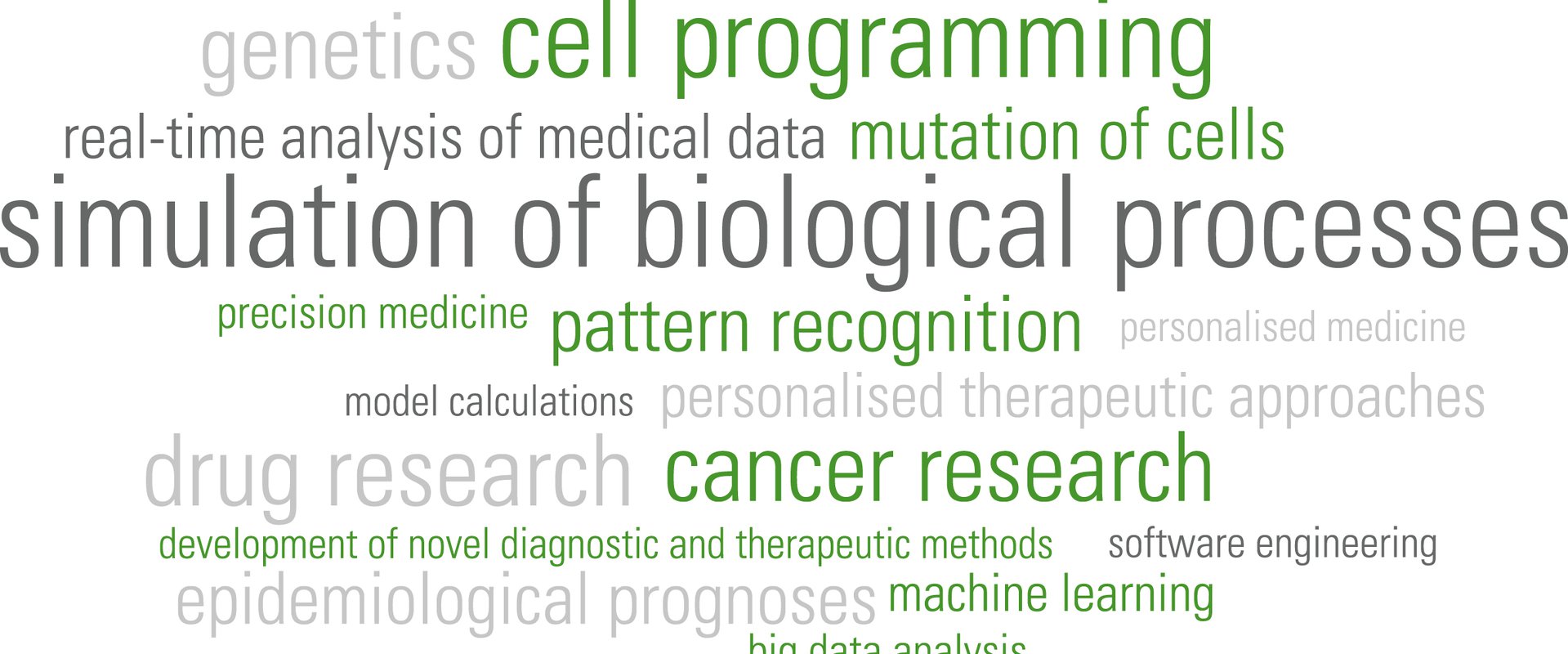
The future of medical research and healthcare is personalized, digitized, and data-driven. The provision, analysis, and interpretation of this data rely on interdisciplinary collaborations. Thus, the foundations for future medical progress are laid at the interface of medicine and computer science.
The field of research and studies Medical Information Sciences has been established as a response to this development, introducing a guest lecture series of the same name in the winter semester of 2022/2023. It adresses current questions from science and provides insights into corresponding areas of industry.
The MIS lecture series will take place this winter semester on Thursdays at 5:30 pm at the Faculty of Applied Computer Science in Lecture Hall N2045. If you are interested in accessing the shared electronic calendar of the lecture series, please send an e-mail to office.bioinf@informatik.uni-augsburg.de.
Additionally, the events will be live-streamed. If you are interested in attending the live-stream, we kindly ask you to register by sending an informal email to office.bioinf@informatik.uni-augsburg.de on time.
The lectures aim at an interested professional audience and will be held in English.
More information about the speakers and their lectures are available on this website or via the official MIS newsletter, which you can register for at the bottom of this webpage.
Continuing Medical Education (CME): All physicians can have two points credited from the "Bayerische Landesärztekammer" (BLÄK, Bavarian General Medical Council) for each individual lecture. Interested physicians can register for participation in advance by sending a message to IDM-Sekretariat@uk-augsburg.de. Participation will be confirmed after the respective event.
In addition, prior to each lecture, we offer an opportunity to discuss individual scientific questions, topics or cooperation opportunites with the speaker. If you are interested, please register in advance by sending a short message to office.bioinf@informatik.uni-augsburg.de.
Below, you find the schedule for the winter semester 2024/2025 with further information on each single lecture:
Location: Lecture hall N2045 (Faculty of Applied Computer Science)
Abstract
Our world is 3D and so is the patient. But visual camera observations are 2D. Advancements in digital imaging now enable capturing rich 3D information of our surrounding, transforming our ability to digitally perceive, present, and analyze data. By combining multiple data sources or frames in a video, we can create context-enhanced digital copies of the physical world as well as the patient - and apply data-driven interpretations and processing. Data sources reach from ceiling mounted cameras in the OR to endoscopic images or robot-mounted sensors. In this talk, we want to look into underlying core ideas of example 3D computer vision pipelines and investigate how these approaches can be used in clinical applications. As a basis for a discussion at this exciting interdisciplinary crossroad, we dive into the subtopics 3D digital reconstruction, data curation for XR, and robot-assisted surgery.
Speaker: Dr. Benjamin Busam
Biography
Benjamin Busam is a Senior Research Scientist with the Technical University of Munich. He coordinates the Computer Vision activities at the Chair for Computer Aided Medical Procedures, I16. Formerly Head of Research at FRAMOS Imaging Systems, he led the 3D Computer Vision & AI Team at Huawei Research, London from 2018 to 2020. Benjamin studied Mathematics at TUM (Germany), ParisTech (France) and at the University of Melbourne (Australia), before he graduated with distinction at TU Munich in 2014. In continuation to a mathematical focus on projective geometry and 3D point cloud matching, he now works on 2D/3D computer vision and sensor fusion and applications into the medical domain. For his work on adaptable high-resolution real-time stereo tracking, he received the EMVA Young Professional Award 2015 from the European Machine Vision Association and was awarded Innovation Pioneer of the Year 2019 by Noah's Ark Laboratory, London. He was given multiple Outstanding Reviewer Awards at 3DV 2020, 3DV 2021, and ECCV 2022.
Location: Lecture hall N2045 (Faculty of Applied Computer Science)
Abstract
Due to the rapid progress in developing experimental techniques, establishing and improving analysis methods is one of the major challenges in computational life sciences. For many analysis tasks, however, the limitations and performance of competing methods remain unknown, and there are no clear rules or guidelines for selecting the optimal analysis method. Benchmark studies have proven to be valuable tools for evaluating the performance and applicability of analysis approaches. However, they are often subject to methodological limitations and deficiencies, leading to potential bias in the results.
In my presentation, I will give an overview of novel approaches developed in my group in the context of mathematical modeling and omics analyses. In particular, I will summarize our ongoing efforts to improve the methodology of benchmark studies. By generally incorporating rigorous planning, design, and analysis principles in benchmark studies, we aim to promote the development of novel analysis approaches and the identification of decision rules for optimal method selection in practice. Especially in view of the enormous efforts to apply deep learning methods in all areas of research, reliable performance comparisons are of great importance.
Speaker: Dr. Clemens Kreutz
Biography
Dr. Clemens Kreutz is the Head of the Methods of Systems Biomedicine (MSB) group and Deputy Director at the Institute of Medical Biometry and Statistics, Medical Center, Faculty of Medicine, University of Freiburg. Since 2018, he has held a permanent position as Group Leader at the institute, and in 2021, he was appointed Deputy Director. Dr. Kreutz's research focuses on the mathematical modeling and analysis of high-throughput data. His work within the field of systems biology has significantly advanced statistical methods for parameter estimation, model selection, and experimental design, contributing to the understanding and modeling of complex biological processes.
Location: Lecture hall N2045 (Faculty of Applied Computer Science)
Abstract
The primary aim of this talk to develop and apply biostatistical methods to improve predictive modeling of data for precision medicine. In order to achieve such a solid framework for different type of datasets the selection of methods are important. However, currently their is no universal method which can be applied for data gathered from humans. To understand the given data at hand, first statistical tools which considers the distribution of the data namely Bayesian posterior distribution will be discussed. Second, to counter one of the most important problems in most of the clinical datasets is the demographics namely matching cohorts for sex would be considered with propensity score matching analyses. In order to model complex datasets, with several input and output variables the structural equation modelling will be delibrated. After understanding and modelling the datasets the prediction will be covered with some machine and deep learning approaches and finally some applications to datasets from multimodal, longitudinal and signal based analyses will be explored. For each methodological aspect an example will be provided with obtained results. The applications will be highlighted with examples and corresponding results. Taken together, the integration of methods leading to the individualized prediction of each subject will be demonstrated.
Speaker: Prof. Muthuraman Muthuraman
Biography
Muthuraman Muthuraman was born in Chennai, India, in 1980. He received the B.E. degree in electronics and communication engineering from the University of Madras, Madras, India, in 2002, and the MS in digital communications in Christian Albrecht’s University, Kiel, Germany in 2006. Ph.D. degree in biomedical engineering from the technical faculty and Department of Neurology of Christian Albrecht’s University, Kiel, Germany, in 2010. In 2010, he joined the Department of Neurology, University of Kiel, as a Post-doc, and in 2013 became a senior post-doc. Since December 2016, he has been with the Department of Neurology, Johannes Gutenberg University Mainz, where he is an Assistant Professor, and the head of the department biomedical statistics and multimodal signal processing unit. Currently from 2024 he is heading the group Informatics for Medical Technology (IMT) in Augsburg as an associate professor and second affiliation to Julius Maximilian university of Würzburg in the department of Neurology and head of the group Neural Engineering with Signal Analytics and Artificial Intelligence (NESA-AI). His current research interests include mathematical methods for time series analysis and source analysis on oscillatory signals, sleep, function of oscillatory activity in central motor systems, biomedical statistics, connectivity analyses, multimodal signal processing and analyses of EEG, MEG, fMRI and EMG, structural and network analyses on anatomical MRI and DTI, functional network analyses on PET imaging, machine learning and deep learning, network analyses on proteomic and genomic data, RNA, mRNA and Spatial transcriptomics.
Location: Lecture hall N2045 (Faculty of Applied Computer Science)
Abstract
Epigenetics, which involves heritable changes in gene expression without DNA sequence alterations, plays a crucial role in tumour classification, with DNA methylation serving as a key biomarker. Tumour classification based on DNA methylation patterns has emerged as a pivotal approach in precision diagnostics, offering insights into cell-of-origin as well as tumour progression. This talk will explore the evolution of methylation-based classifiers, with a focus on CNS tumours, and highlight recent advances in using methylation profiling to achieve accurate and scalable diagnostics. Additionally, the talk will introduce development and application of novel technologies like nanopore sequencing and AI-based computational histopathology to improve accessibility of cutting-edge molecular diagnostics.
Speaker: Areeba Patel
Biography
Areeba Patel is a postdoctoral researcher at the German Cancer Research Center (DKFZ) affiliated to the departments of Neuropathology, Paediatric Neurooncology and AI in Oncology. She completed her masters in Cancer Biology at Imperial College London followed by PhD in computational neuro-oncology at the DKFZ. Her research revolves around developing novel solutions using nanopore sequencing as well as AI-based computational histopathology to make precision CNS tumour diagnostics swift, accessible and affordable.
Location: Lecture hall N2045 (Faculty of Applied Computer Science)
Abstract
In his talk, he will explore how computer vision tools are impacting the analysis of movement disorders and their potential going forward. In particular, he will discuss recent work on tracking the head movements of cervical dystonia patients to automate clinical scoring and identify novel kinematic biomarkers. Finally, he will also introduce some novel approaches for analysing whole-brain EEG data via the vector fields of phase-gradients.
Speaker: Dr. Robert Peach
Biography
Robert Peach is an independent research group leader at the University Hospital Würzburg's Neurology Department and a Senior Research Fellow in the Department of Mathematics at Imperial College London. He earned his Ph.D. in Computational Biology from Imperial College London, where he explored protein dynamics using graph theory and single-molecule spectroscopy. His current research stretches from mathematics to neuroscience with a focus on applications in movement disorders. In particular, he specialises in using and developing methods in computer vision, geometry, and machine learning to automate the analysis of movement disorders, both in the kinematic and neural measurement domains. In his talk, he will explore how computer vision tools are impacting the analysis of movement disorders – with various examples - and their potential going forward. Finally, he will also introduce some novel approaches for analysing EEG data.
Location: Lecture hall N2045 (Faculty of Applied Computer Science)
Abstract
Bioimpedance analysis is an established non-invasive method that is already in clinical use for body composition measurement or electrical impedance tomography. However, novel instrumentation and signal processing approaches enable significant improvements of these applications as well as new measurement approaches. This presentation will give a brief insight into the theory of bioimpedance analysis and discuss instrumentation approaches. Subsequently, examples of new scientific measurement approaches will be presented. The focus will be on the detection of skeletal muscle contractions (impedance myography) and pulse wave detection (impedance plethysmography).
Speaker: Prof. Dr. Roman Kusche
Biography
Roman Kusche has been a Professor in the Department of Computer Science at HAW Hamburg since 2023, where he leads the Medical Sensors Lab. His research interests include the development of novel biomedical measurement methods and related medical electronic devices. Before joining HAW Hamburg, he was the Head of the Department for Individualized Therapy and led the Medical Electronics research group at Fraunhofer IMTE. Roman Kusche has a background in electrical engineering and received his Ph.D. from the Institute of Medical Engineering at the University of Lübeck.
Location: Lecture hall N2045 (Faculty of Applied Computer Science)
Abstract
Artificial Intelligence (AI) is transforming our world and society with extraordinary speed. What does this mean for biomedical research and disease detection? What opportunities and risks arise from this? In my talk, I will present examples from the biomedical and clinical research conducted by my research group at the Helmholtz Center Munich. I will cover the fundamentals of machine learning and AI and showcase applications that allow individual cells to be characterized with unprecedented accuracy. These technological breakthroughs promise new perspectives for both basic research and the personalized medicine of the future.
Speaker: Dr. Carsten Marr
Biography
Carsten Marr is the founding director of the Institute of AI for Health at Helmholtz Munich, a European center for applied artificial intelligence. His goal is to develop AI-based methods to improve the diagnosis, treatment, and understanding of diseases. After studying theoretical physics at the Technical University of Munich and completing his diploma thesis at the Max Planck Institute of Quantum Optics, Carsten switched from physics to theoretical biology. His PhD thesis at Technical University of Darmstadt focused on the architecture of biological networks and was awarded the best of its year in the Department of Biology. After postdoctoral research stays in Munich, Bremen and Edinburgh, he started his research group at the Helmholtz Munich in 2013 and became deputy head of the Institute of Computational Biology. In interdisciplinary projects with experimentalists, biomedical experts, and clinicians, he pioneered the training of deep neural networks on life science data for the prediction of stem cell decisions from microscopic images and the identification of leukemia from blood and bone marrow smears. He has received several awards for his research and analysis of single cell data as well as an ERC Consolidator Grant for the training of AI models for the automated analysis of blood diseases.
Location: Lecture hall N2045 (Faculty of Applied Computer Science)
Abstract
Sleep disorders represent a critical global health challenge, significantly affecting physical and mental health while imposing considerable economic burdens. Polysomnography (PSG) remains the diagnostic gold standard, but it is highly resource-intensive, leading to long waiting times and widespread underdiagnosis. Innovative technologies focusing on wearable and contactless monitoring have emerged to address these shortcomings, offering noninvasive, cost-effective alternatives for sleep analysis. This presentation investigates advancements in contactless sleep monitoring. This technology leverages techniques like movement analysis to measure cardiorespiratory parameters such as heart rate and respiration. It aims to provide accurate diagnostics without physical contact. The study highlights ongoing research into AI-driven solutions that integrate noninvasive sensors, machine learning algorithms, and user-friendly interfaces to identify sleep stages and detect disorders like apnea and insomnia. These approaches demonstrate promising accuracy in monitoring vital signs and diagnosing conditions, showcasing the potential to bridge the gap between clinical-grade diagnostics and home-based monitoring. Efforts to refine these technologies, such as improving data scalability and reducing development costs, aim to make sleep monitoring more accessible and effective. AI-powered systems also enable automated sleep stage scoring, combining traditional signal processing techniques with advanced machine learning to deliver efficient, objective analyses of physiological data. The findings underscore the transformative potential of contactless sleep monitoring technologies in advancing sleep medicine. By enhancing accessibility and diagnostic efficiency, these innovations promise to improve individuals' health outcomes while addressing the systemic limitations of conventional diagnostic methods.
Speaker: Prof. Ralf Seepold
Biography
Prof. Dr. Ralf Seepold is Professor and Chair for Ubiquitous Computing at HTWG Konstanz (Germany), Director of the Ubiquitous Computing Lab and the Interdisciplinary Sleep Lab. His research fields are non-invasive physiological measurement, medical IoT, sleep medicine, ubiquitous AI, and aging society. Prof. Seepold is a Co-opted Professor at the University of Seville (Spain) in the Faculty of Computer Engineering. He is a visiting professor at the Southern Taiwan University of Science and Technology and was a visiting professor of Digitalization in Medicine at the Institute of Digital Health at I.M. Sechenov First Moscow State Medical University in Russia. He is currently leading the research initiative “ReSiliente Systeme (ReSiS) Strengthening resilience in complex systems” at HTWG. Prof. Seepold is “IEEE Senior Member”, a member of the “German Society for Biomedical Technology (DGBMT)”, a member of the European Sleep Research Society (ESRS), and a member of the Telemedicine Working Group of the German Sleep Research Society (DGSM).
Location: Lecture hall N2045 (Faculty of Applied Computer Science)
Abstract
scATAC-seq analysis usually relies on peak feature spaces to build chromatin profiles and distinguish cell types. Peaks correspond to genomic sequences particularly open in the genome, their differential openness allows to identify what is usually considered a cis-regulatory sequence. Another popular approach is to measure the chromatin openness of genes as a proxy for gene expression, also called gene activity. While these are powerful approaches to describing the chromatin profiles of cell types, they fail to describe the epigenomic regulation of non-coding and repetitive elements of the genome, such as LINEs, tRNAs, rRNAs, etc. These genomic annotations are experimentally difficult to measure and usually require computational approaches specific to the type of feature being investigated. Here, we developed a novel pipeline that reveals the annotation-specific chromatin profiles of different cell types using scATAC-seq data. The pipeline tackles the issues of multi-mapping, extreme sparsity of sequencing, and overlapping annotations, which otherwise hinder a successful quantification of the different annotation chromatin profiles. We tested the pipeline on multiple scATAC-seq human PBMC samples, identifying similar profiles for tRNAs, rRNAs, L1-LINEs, etc. both at the level of the annotation sub-families and in a loci-specific manner. Our results reproduce some already described tRNA and L1-LINEs expression and regulation patterns and identify more cell type specific factors that we are currently validating experimentally to determine their effect on cell identity and cell function.
Speaker: Anna Danese, M.Sc.
Biography
Anna Danese is a researcher specializing in reprogramming and regeneration at the Biomedical Center (BMC), Physiological Genomics, Faculty of Medicine, Ludwig Maximilian University (LMU) Munich. She is also involved in epigenetic engineering at the Institute of Stem Cell Research, Helmholtz Zentrum, German Research Center for Environmental Health.
Location: Lecture hall N2045 (Faculty of Applied Computer Science)
Abstract
Wearables have been the dominant consumer monitoring device for the past decades, however, not the ideal one. Battery dependency, correct placement, good contact with skin, etc., all impose requirements and restrictions that can’t be met by everyone (e.g., vulnerable populations, skin conditions, etc.). In light of this and in accordance with the ultimate idea of ubiquitous computing, the interaction between the user and sensor should be minimized or ideally removed entirely. This is being achieved with improvements and availability of sensors – namely RGB cameras, thermal cameras, radars, LIDARs, and others – accompanied by immense development of machine learning, specifically deep neural networks. In this talk we will overview existing methods for unobtrusive contact-free monitoring of physiological parameters and some psychological states that can be derived from them.
Speaker: Dr. Gašper Slapničar
Biography
Dr. Gašper Slapničar is the head of the ambient intelligence group at the department of intelligent systems, Jožef Stefan Institute. He finished his undergrad studies in computer science from the faculty of computer and information science, university of Ljubljana, and then pursued his PhD at Jožef Stefan international postgraduate school, focusing on contact-free physiological monitoring using radar and optical sensors. He is the author of over 30 scientific papers, many published in impact factor journals and at established conferences such as ICCV and BHI. He is also a regular program committee member for the computer vision for physiological measurement (CVPM) workshop. His ongoing research includes unobtrusive contact-free monitoring of complex states, including emotions and well-being.
Subscription to the MIS newsletter
We appreciate your interest in the work of the Chair of Biomedical Informatics, Data Mining and Data Analytics!
Filling in the following registration form, you can request to receive regular updates on current information as well as notifications of events related to the MIS field of research.
Registration form
Submission successful
Many thanks
By subscribing to our media, you agree to the processing of your data for the purpose of delivering the selected media, as well as to the data protection policies of the University of Augsburg. The collected data is solely used for delivering the selected media and documenting your consent. There is no other processing of the data and no sharing of the data with third parties. You may revoke your subscription and consent to the storage of your data at any time without giving any reasons. Please send a simple email to office.bioinf@informatik.uni-augsburg.de for this purpose.