Datasets
Haystack Panoptic Scene Graph Dataset
Available soon.
Jump-Broadcast Dataset
This dataset consists of images from 26 publicly available videos of triple, high, and long jump competitions on YouTube. 9 videos cover triple jump competitions, 8 videos long jump competitions and the remaining 9 videos high jump competitions. 193 different male and female athletes are present in the video footage. This dataset contains keypoint annotations made by hand and segmentations masks that are only partly correct and automatically retrieved. The dataset contains 2403 annotated images and is split into train/test/val subsets with 1805, 576 and 122 images, resprectively. The train and validation annotations are included in the download package. If you are interested in the test annotations as well, contact Katja Ludwig via E-Mail.
The annotation files of the dataset include the following information:
- The name of the athlete, retrieved from the video footage.
- A flag indicating if the image belongs to a slowmotion replay or not.
- 2D image keypoint coordinates from head, neck, r. shoulder, r. elbow, r. wrist, r. hand, l. shoulder, l. elbow, l. wrist, l. hand, r. hip, r. knee, r. ankle, r. heel, r. toe tip, l. hip, l. knee, l. ankle, l. heel, l. toe tip and their visibility (0: invisible, 1: occluded, 2: visible)
Segmentation masks
The dataset further contains 1809 segmentation masks. They are automatically generated as described in our paper. These segmentation masks are only partly correct. This means that the segmentations for some body parts are wrong, incomplete or missing. The following body parts are included in the segmentation masks: head, torso, l./r. upper arm, l./r. forearm, l./r. hand, l./r. thigh, l./r. lower leg, l./r. foot
License
If you are using this dataset, please cite the following paper
@InProceedings{ludwig2023all_kps,
title = {All Keypoints You Need: Detecting Arbitrary Keypoints on the Body of Triple, High, and Long Jump Athletes},
author = {Ludwig, Katja and Lorenz, Julian and Sch{\"o}n, Robin and Lienhart, Rainer},
booktitle = {Proceedings of the 2023 IEEE/CVF International Conference on Computer Vision and Pattern Recognition Workshops (CVPRW)}},
month = {June},
year = {2023},
}
Download
You can download the dataset here: jump-broadcast.zip
If you have any questions or want access to the test set annotations, contact Katja Ludwig
YouTube Skijump Dataset
This dataset consists of images from 10 publicly available videos of skijump competitions on YouTube. It contains keypoint annotations made by hand and some segmentation masks that are only partly correct and mostly automatically retrieved. The dataset contains 2867 annotated images and is split into train/test/val subsets with 2159, 560 and 148 images, resprectively. The train and validation annotations are included in the download package. If you are interested in the test annotations as well, contact Katja Ludwig via E-Mail.
The annotation files of the dataset include the following information:
- The name of the athlete, retrieved from the video footage.
- A flag indicating if the image belongs to a slowmotion replay or not.
- 2D image keypoint coordinates from head, r. shoulder, r. elbow, r. hand, l. shoulder, l. elbow, l. hand, r. hip, r. knee, r. ankle, l. hip, l. knee, l. ankle, r. ski tip, r. ski tail, l. ski tip, l. ski tail and their visibility (0: invisible, 1: occluded, 2: visible)
The dataset splits are created such that each athlete is only contained in one split.
Segmentation masks
The dataset further contains 424 segmentation masks. Most of them are automatically generated as described in our paper. These segmentation masks are only partly correct. This means that the segmentations for some body parts are wrong, incomplete or missing. The following body parts are included in the segmentation masks: head, torso, l./r. upper arm, l./r. forearm, l./r. hand, l./r. thigh, l./r. lower leg, l./r. foot, l./r. ski
License
If you are using this dataset, please cite the following paper
@InProceedings{ludwig2023arbitrary_kps,
title = {Detecting Arbitrary Keypoints on Limbs and Skis with Sparse Partly Correct Segmentation Masks},
author = {Ludwig, Katja and Kienzle, Daniel and Lorenz, Julian and Lienhart, Rainer},
booktitle = {Proceedings of the IEEE/CVF Winter Conference on Applications of Computer Vision (WACV)},
month = {January},
year = {2023},
}
Download
You can download the dataset here: youtube_skijump_dataset.zip
If you have any questions or want access to the test set annotations, contact Katja Ludwig
DensePose
These files provide 3515 left-right annotation corrections for the DensePose subset of the MSCOCO-Dataset. Some of these corrections were made per hand, others according to heuristics. Therefore, the dataset might still contain some small errors. You will find corrections for upper arm, lower arm, thigh and lower leg, other bodyparts were not considered. The indices in the files correspond to the following standard bodypart indices used in the DensePose data:
1: Torso, 2: Right Hand, 3: Left Hand, 4: Left Foot, 5: Right Foot, 6: Upper Leg Right, 7: Upper Leg Left, 8: Lower Leg Right, 9: Lower Leg Left, 10: Upper Arm Left, 11: Upper Arm Right, 12: Lower Arm Left, 13: Lower Arm Right, 14: Head
The files contain the MSCOCO image id, the person number (in the order of the MSCOCO annotations in the original *.json files, 0-indexed) and the ids of the body parts to be swapped according to the list.
Downloads:
densepose_coco_2014_train1_corrections.csv
densepose_coco_2014_train2_corrections.csv
densepose_coco_2014_val_corrections.csv
If you have any questions, contact Katja Ludwig
FlickrLogos
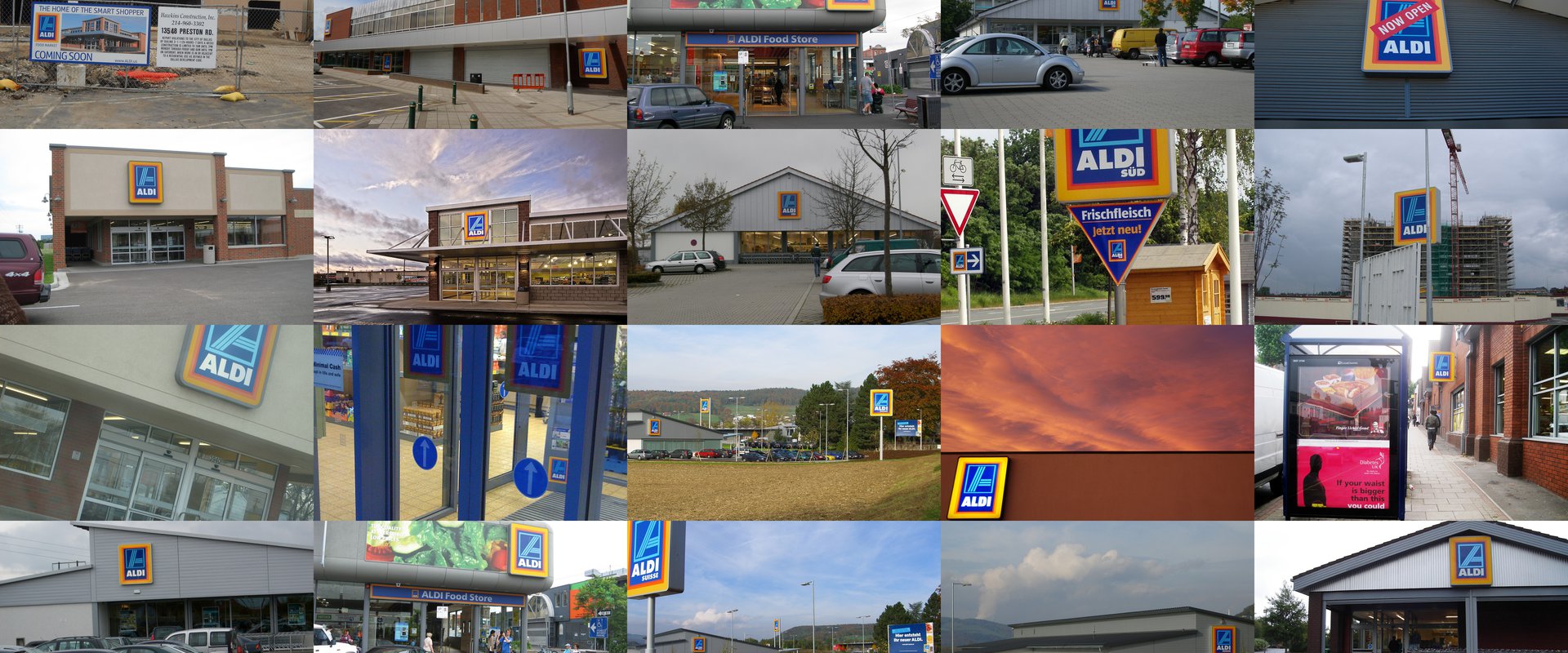
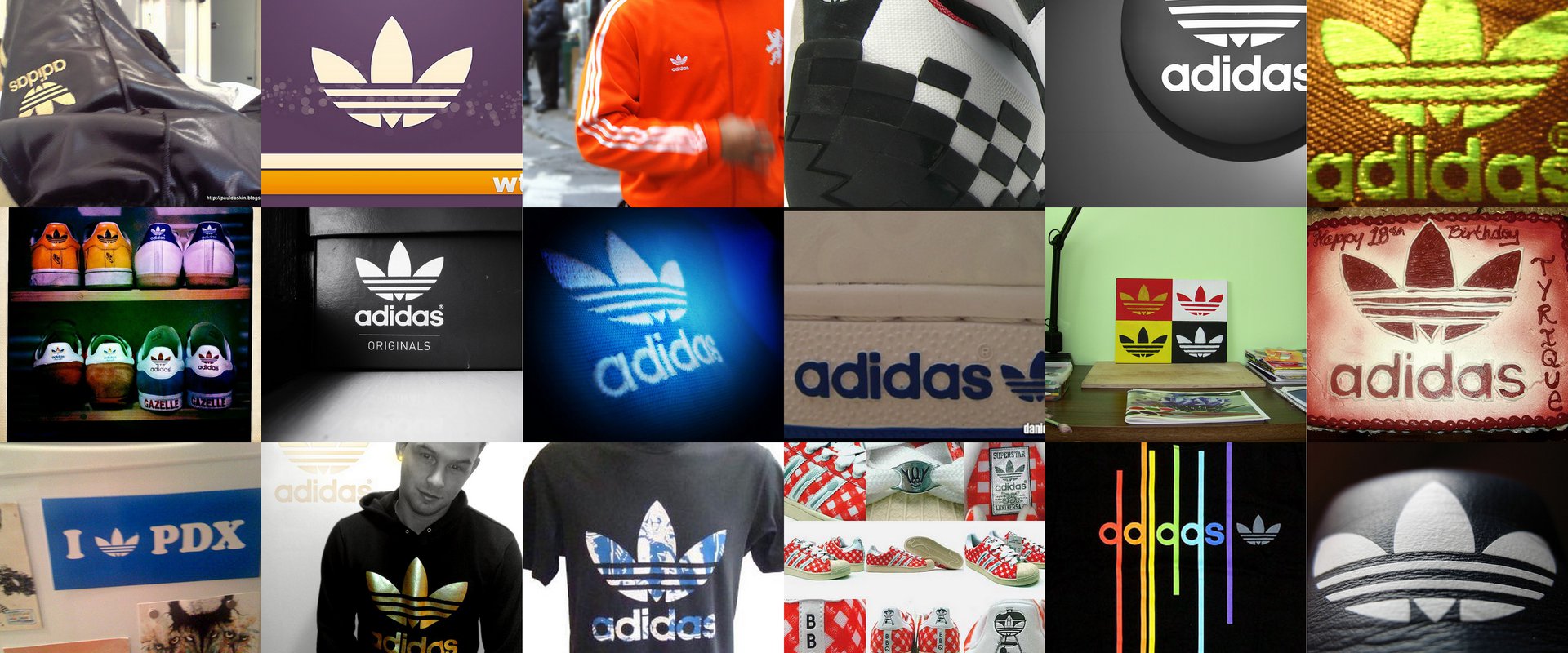
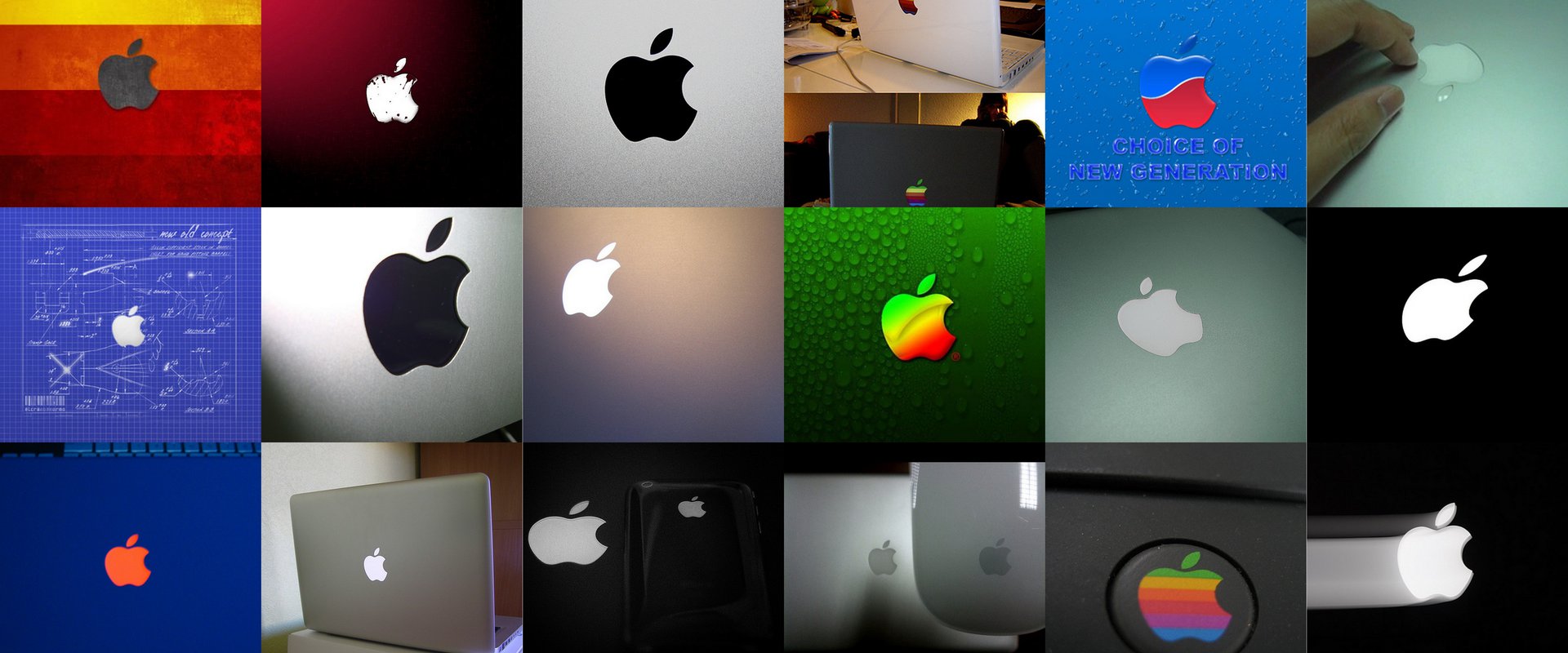
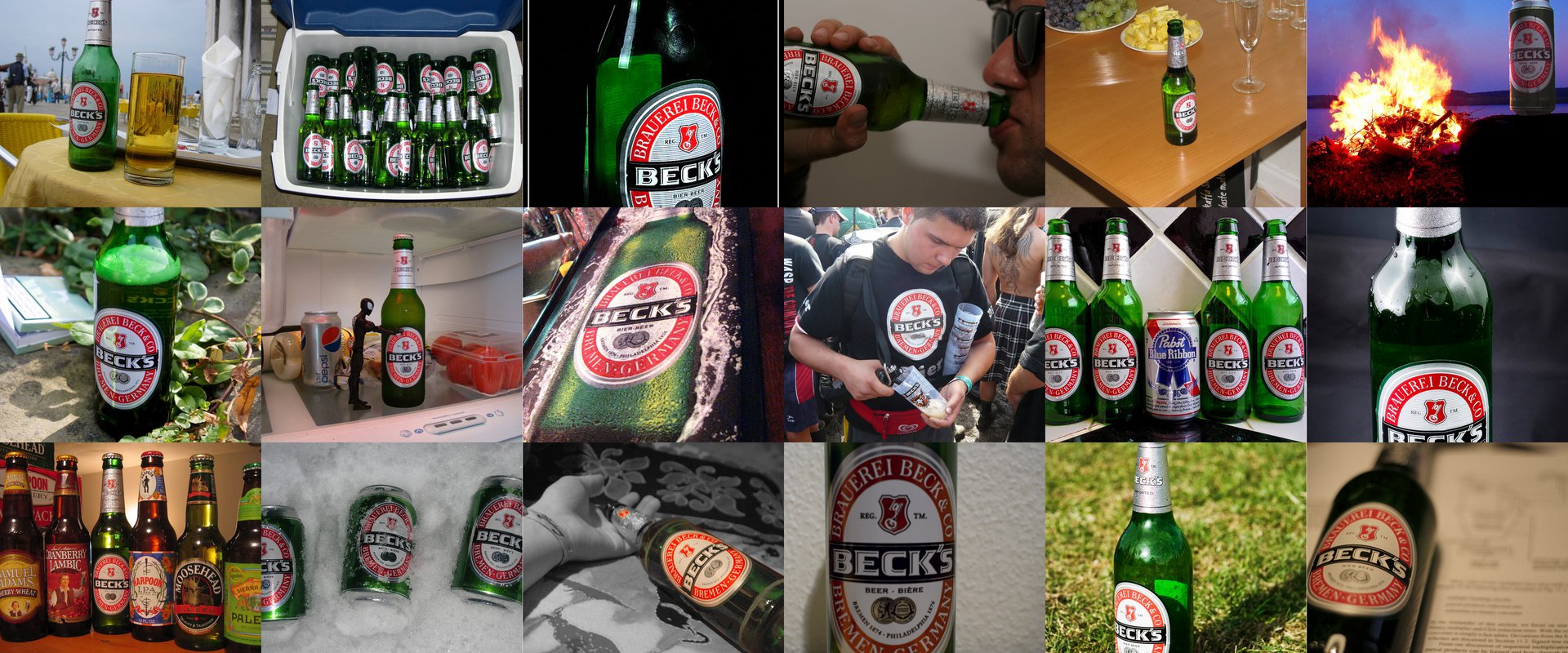
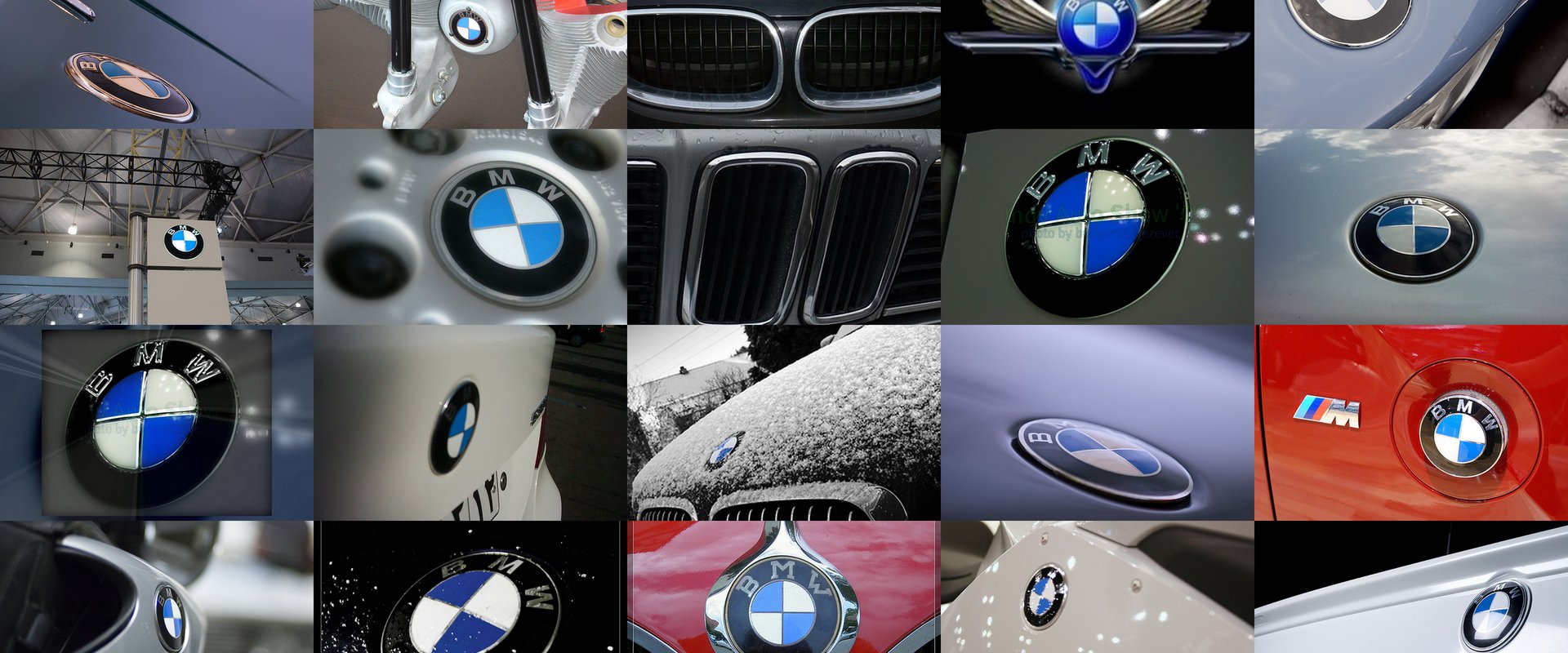
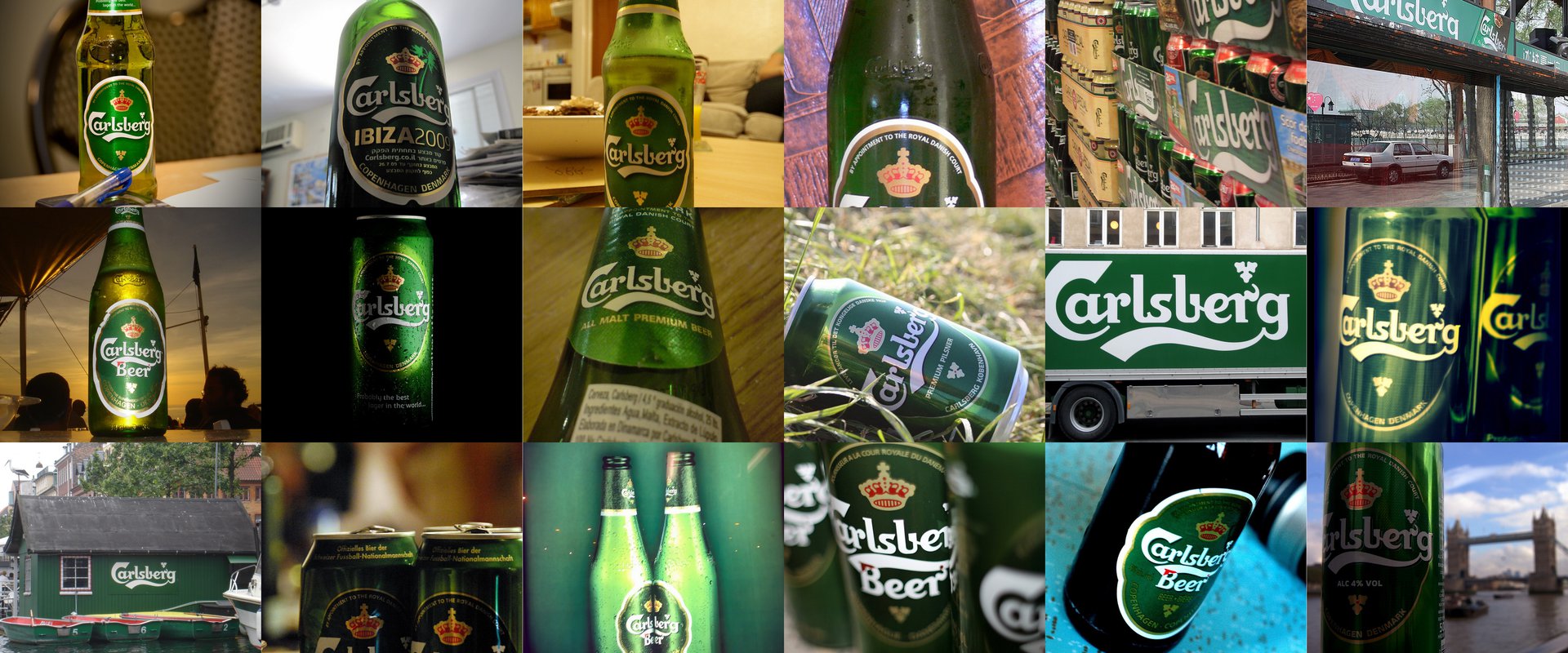
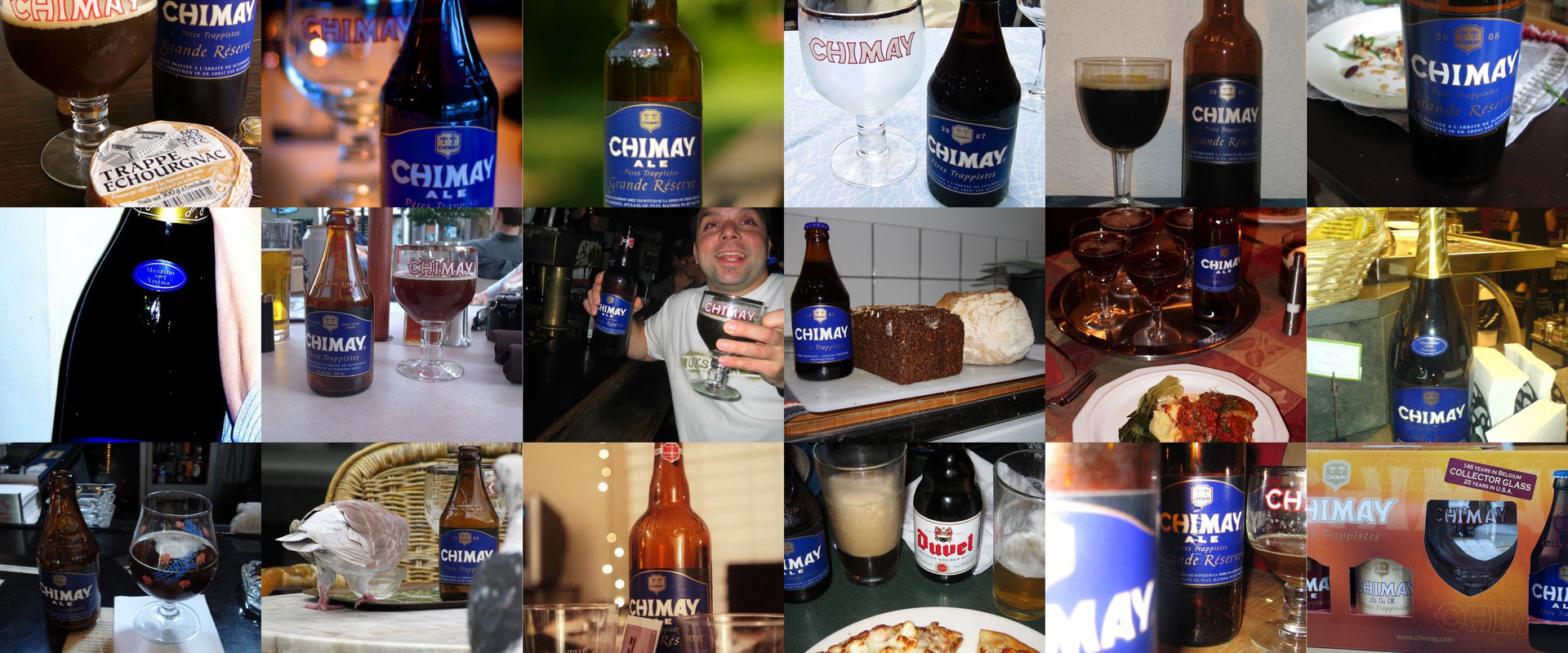
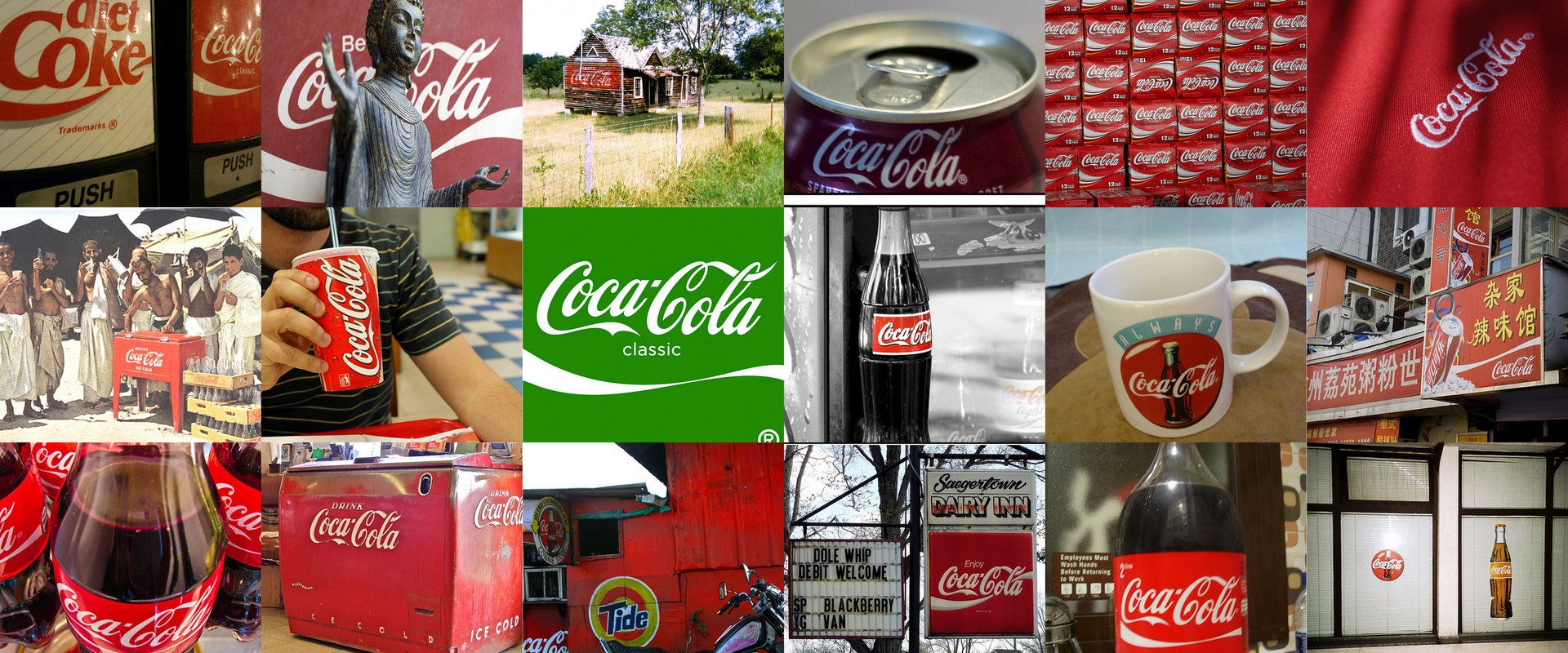
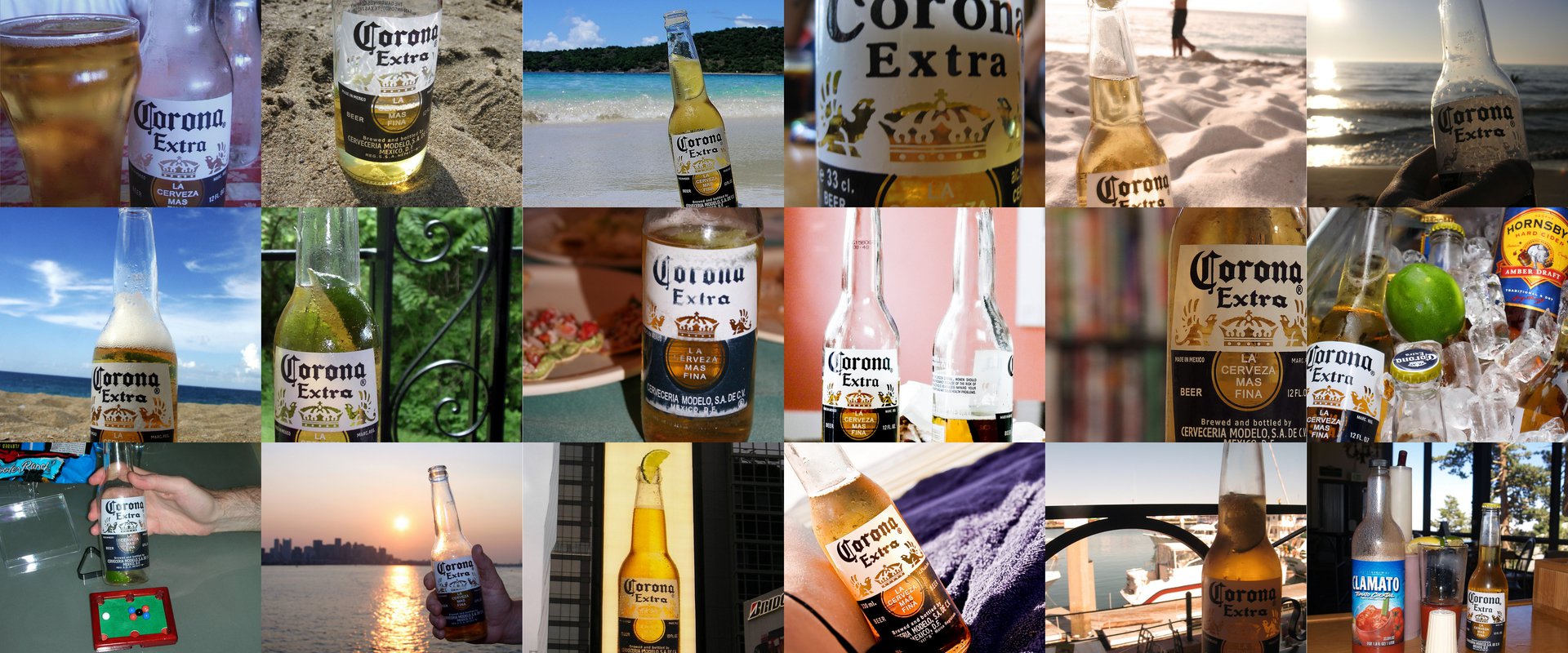
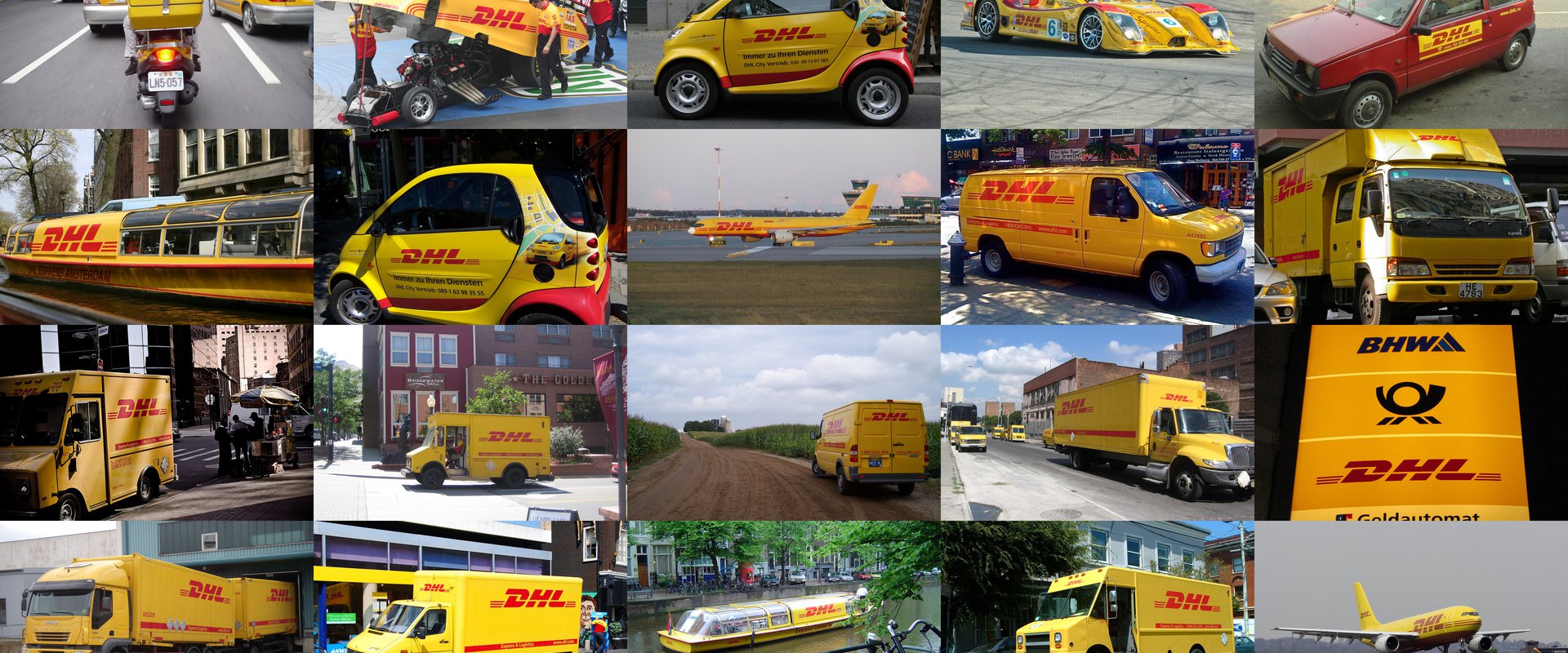
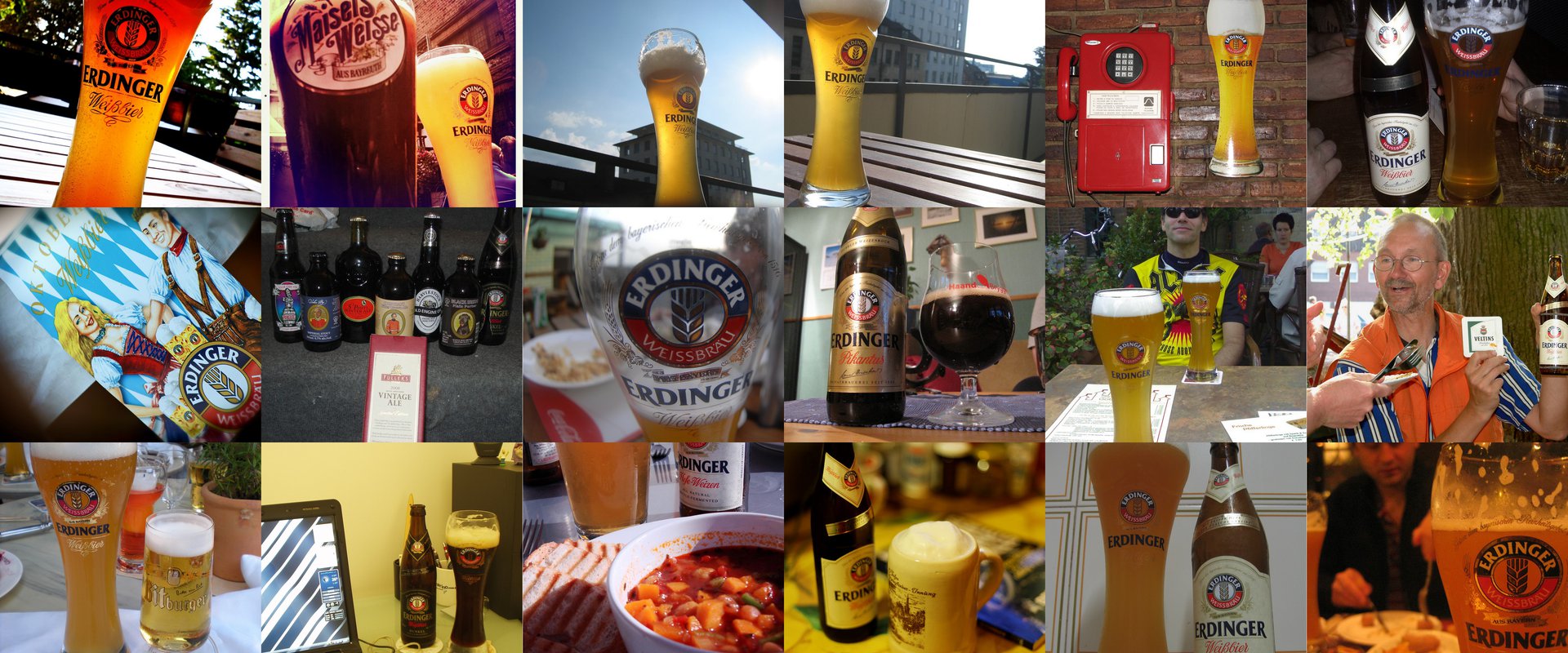
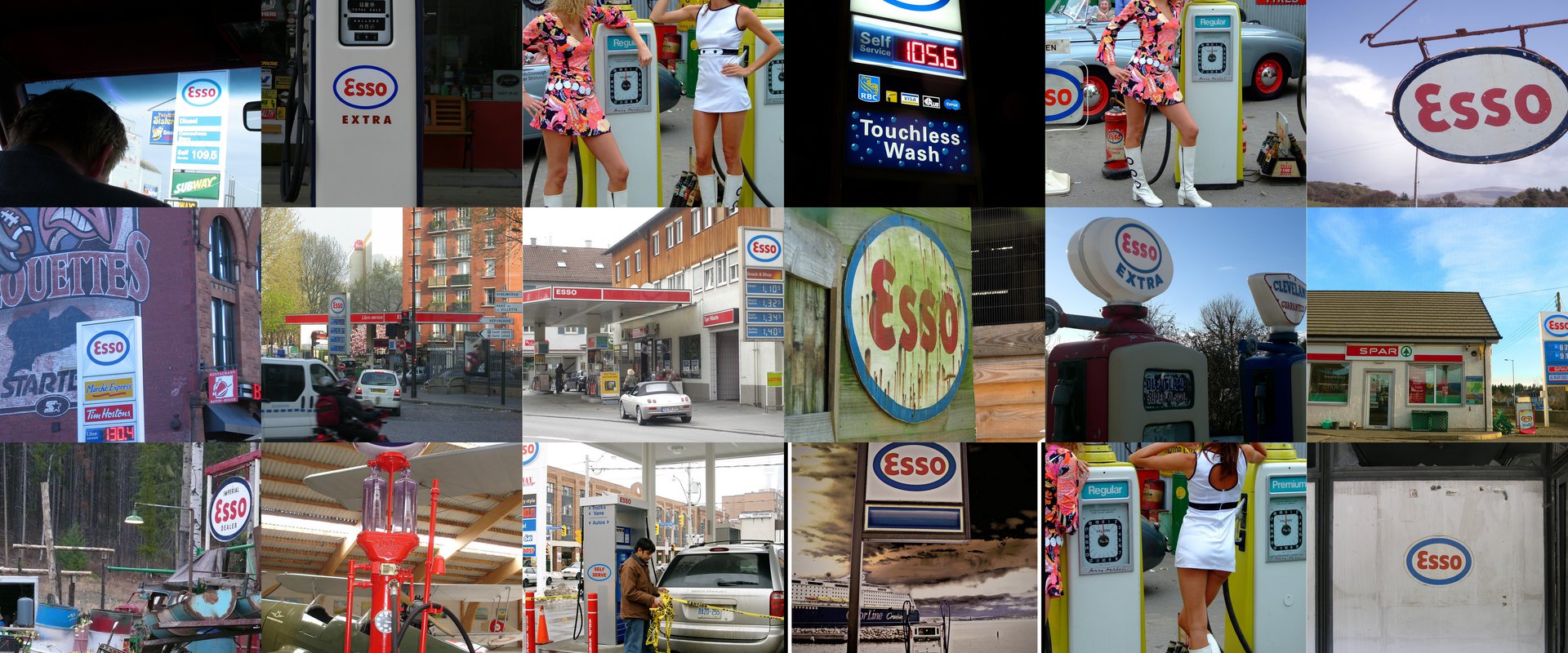
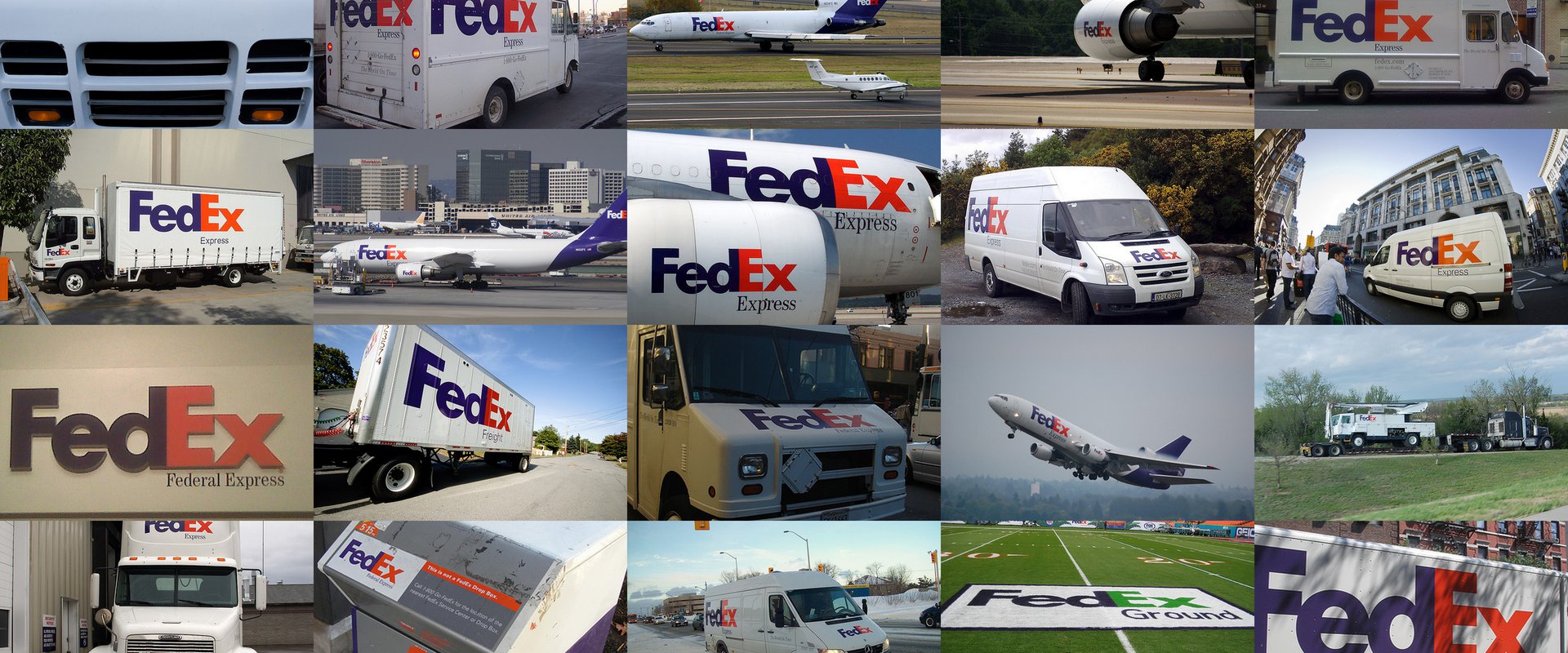
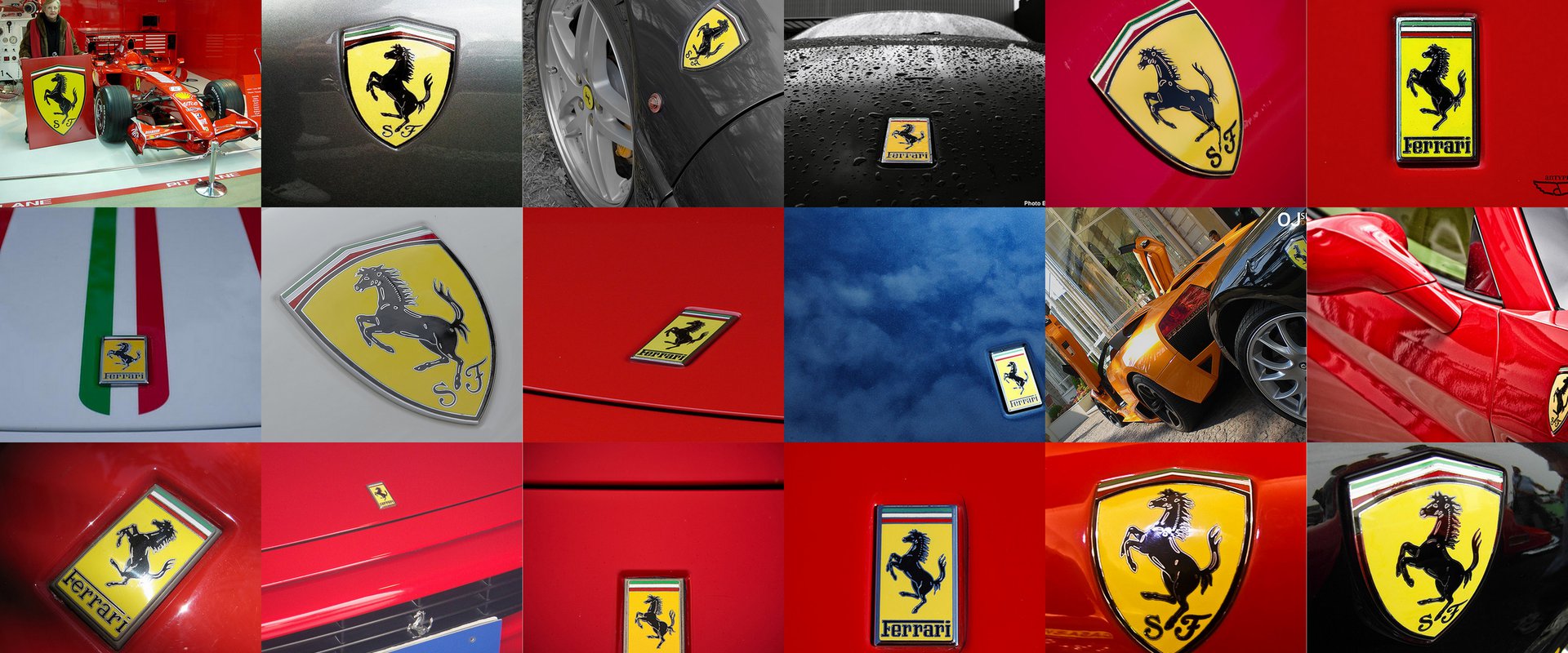
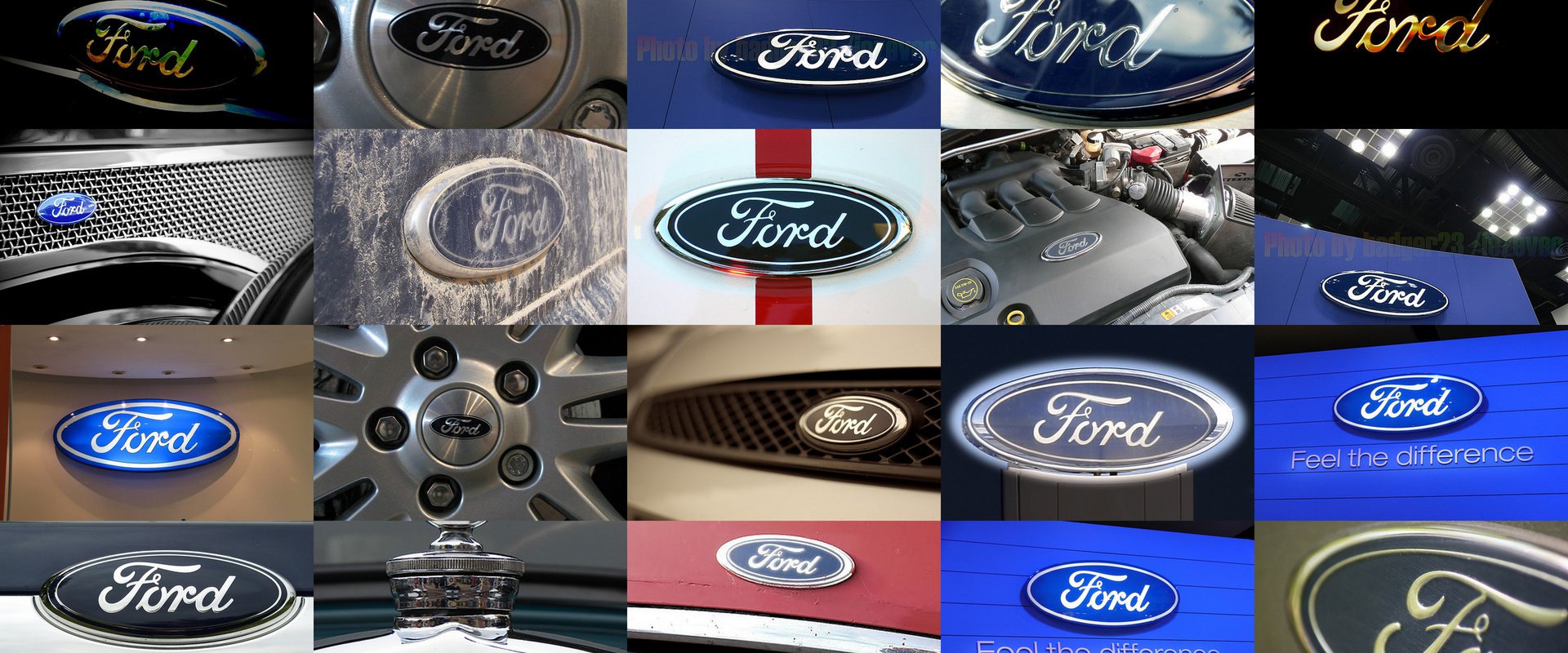
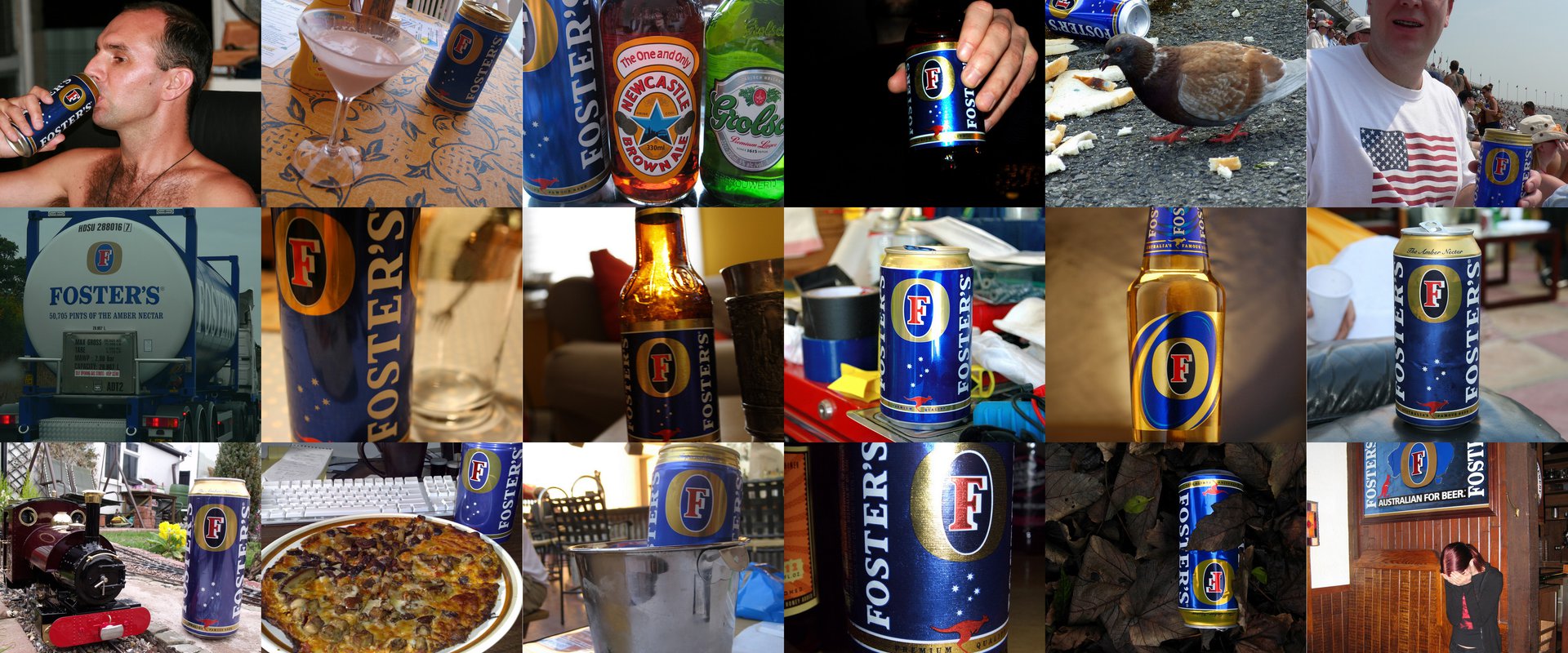
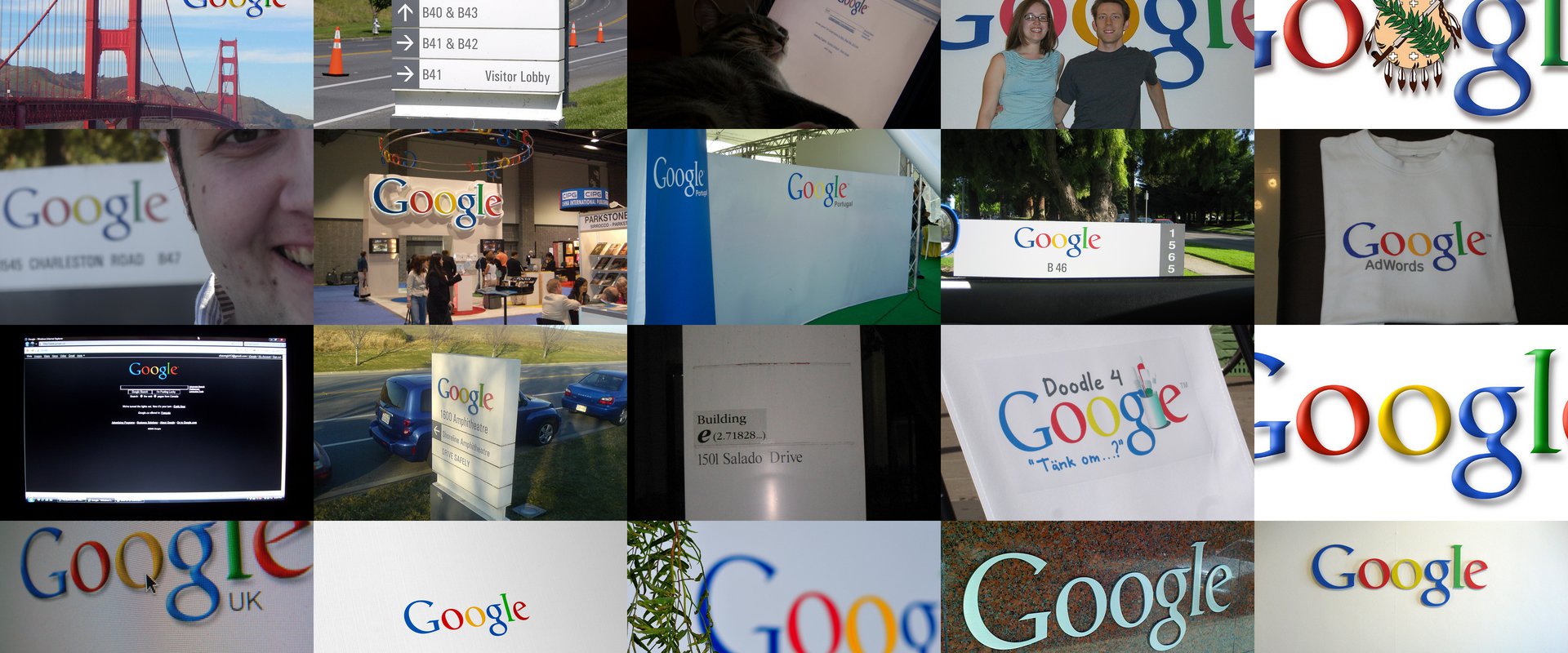
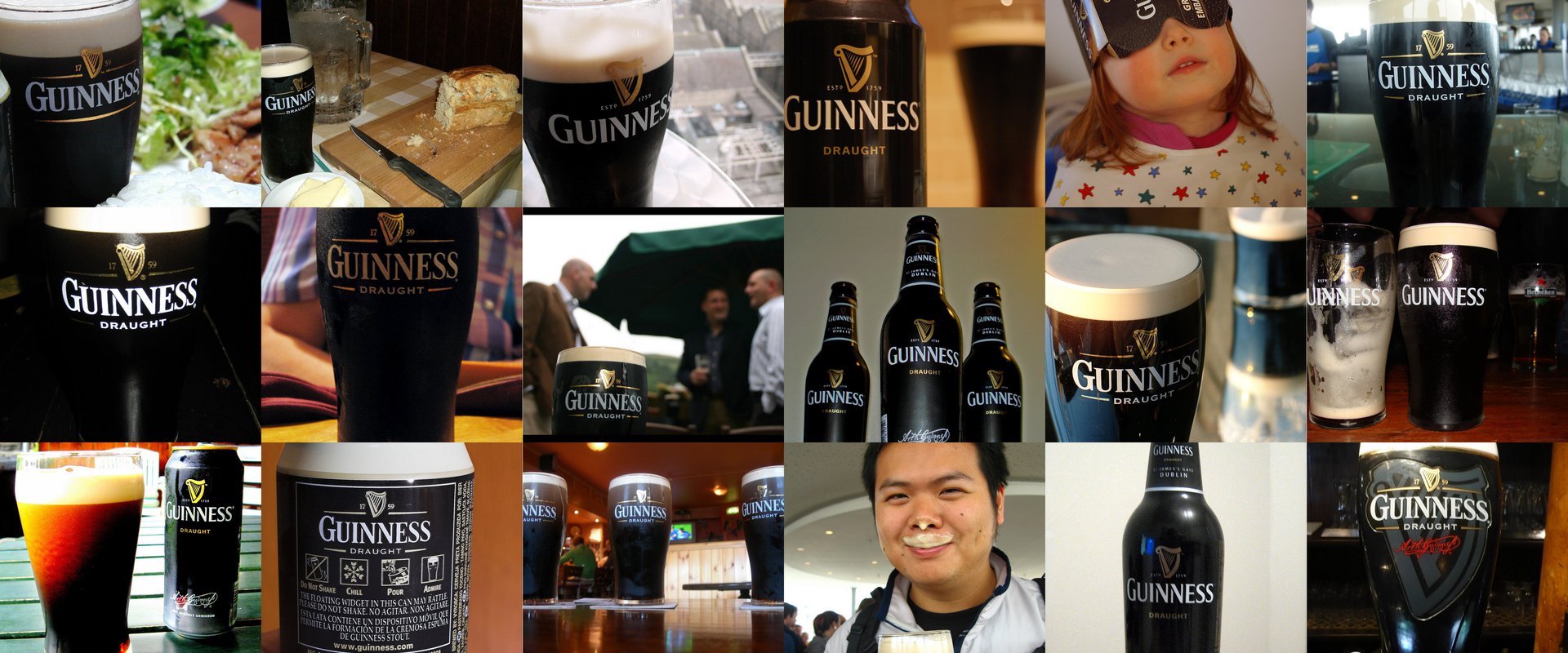
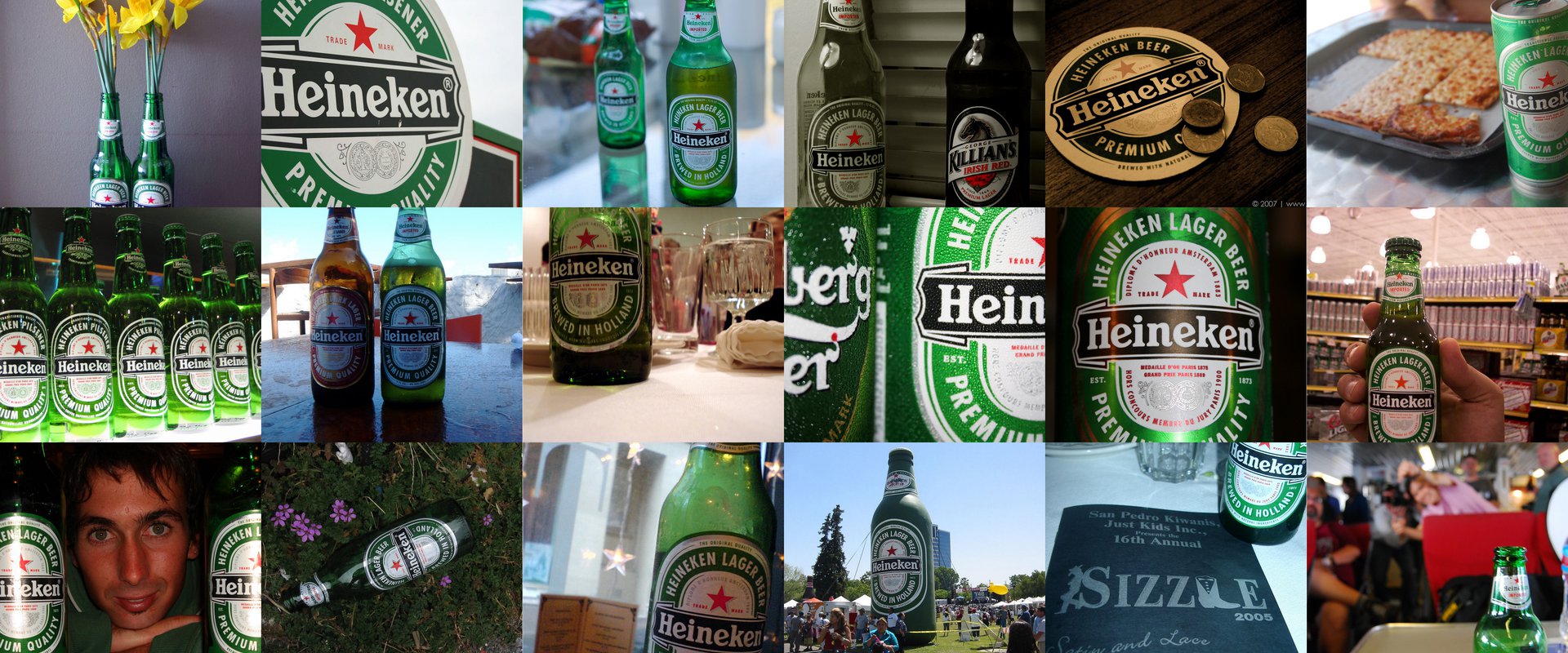
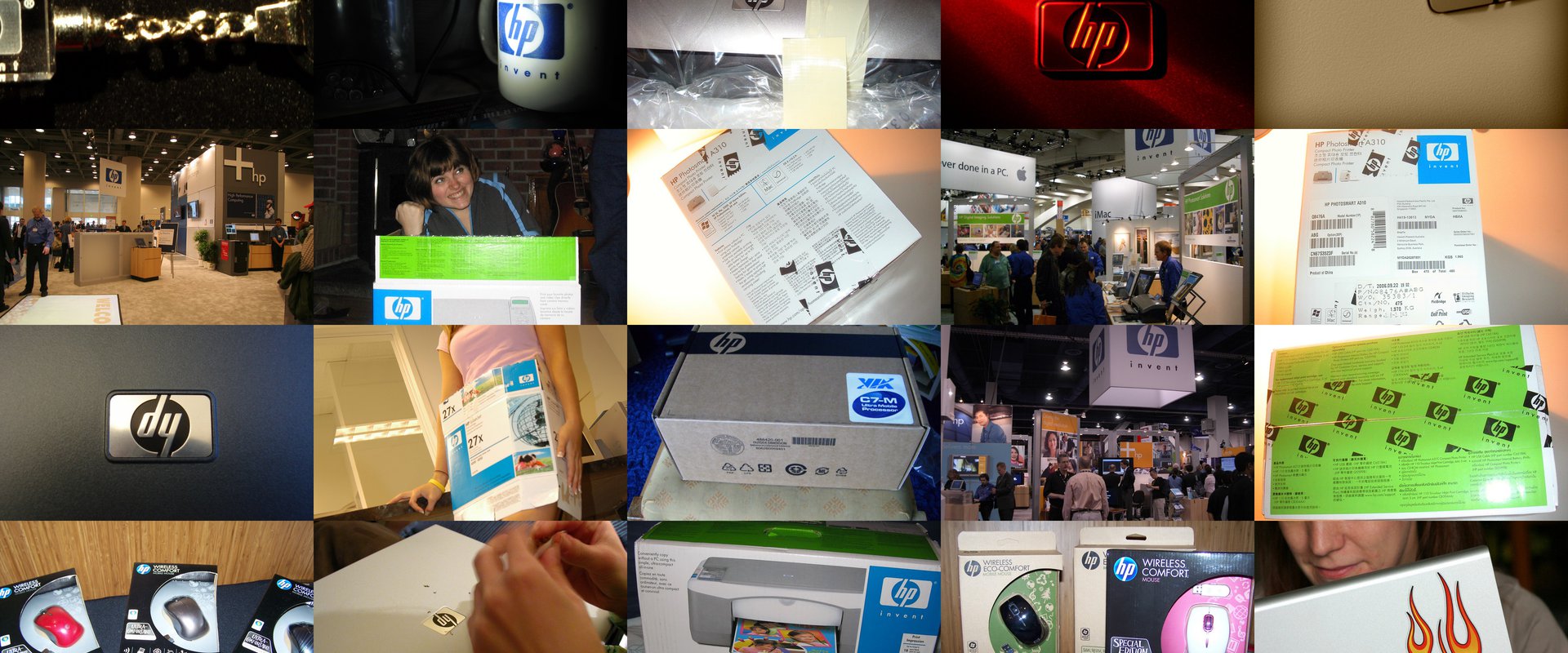
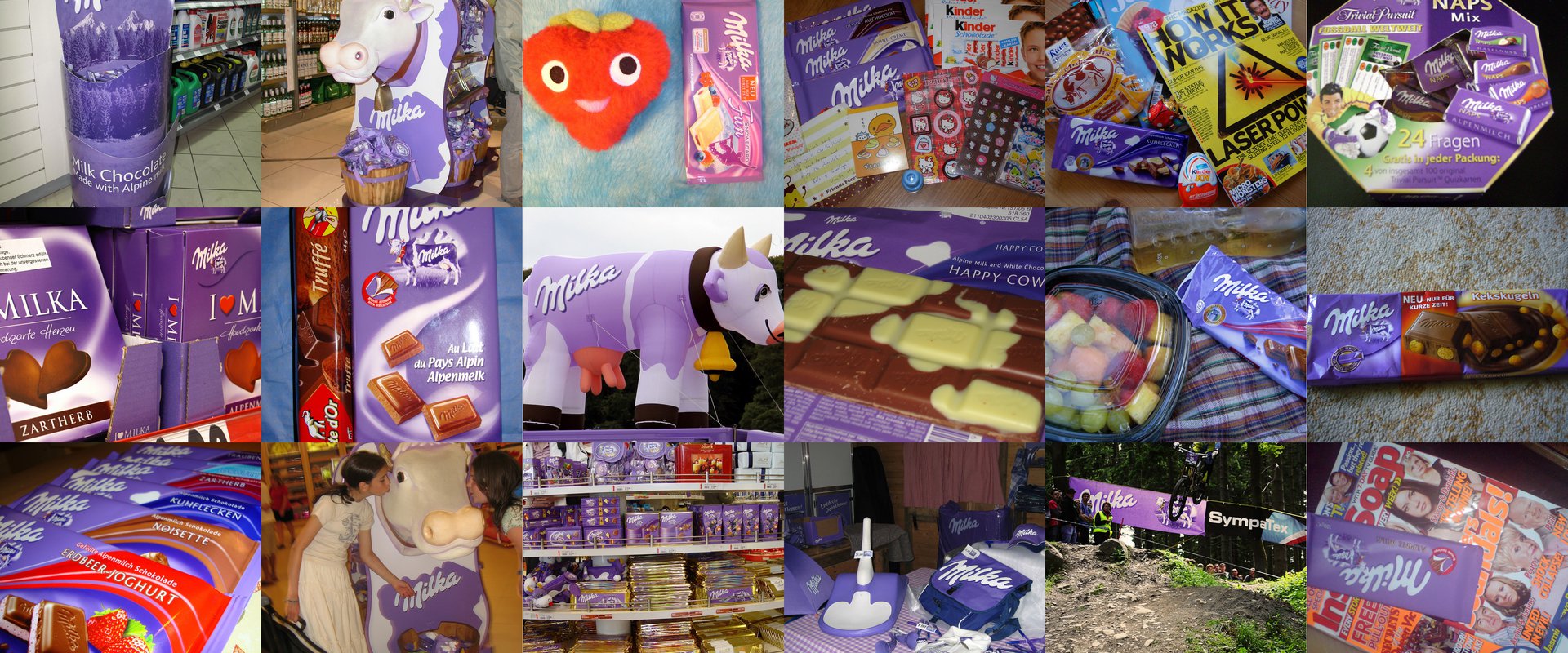
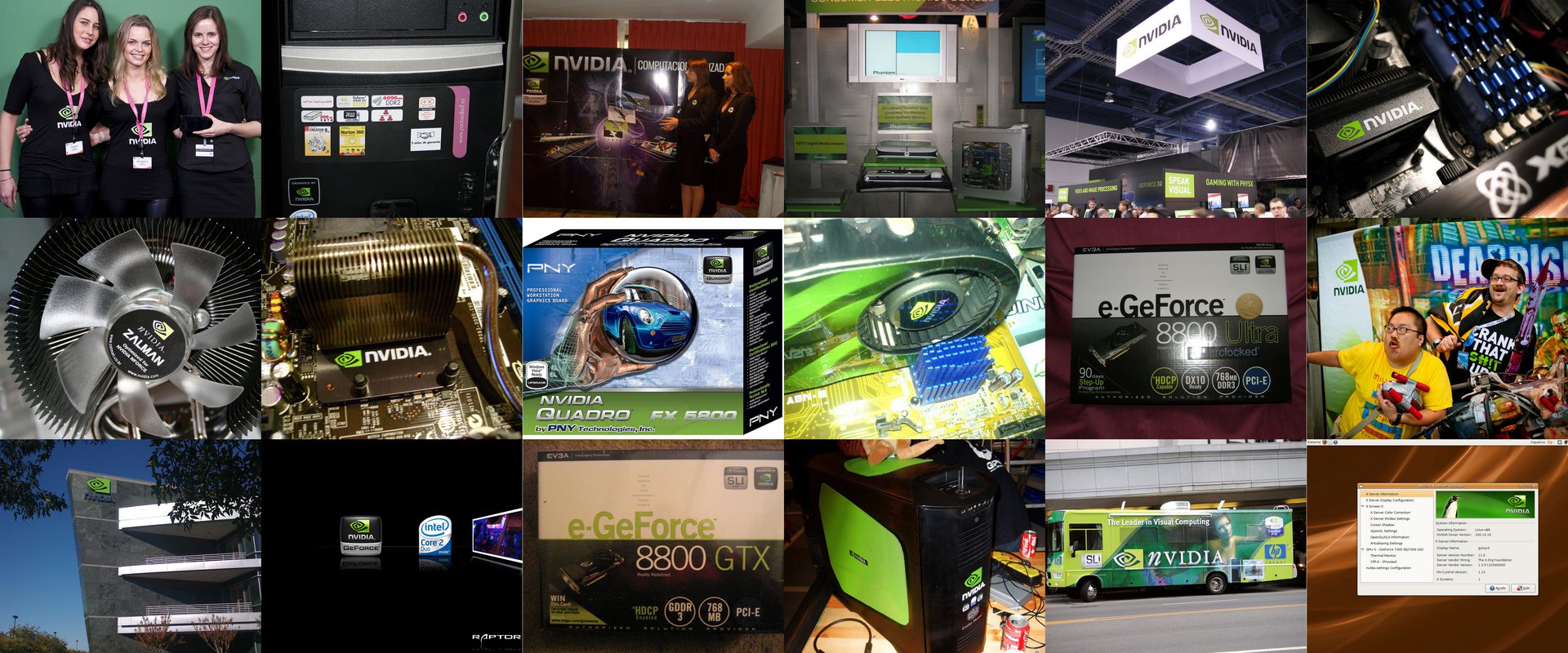
The FlickrLogos dataset consists of real-world images collected from Flickr depicting company logos in various circumstances. The dataset comes in two versions: The original FlickrLogos-32 dataset and the FlickrLogos-47 dataset. FlickrLogos-32 was designed for logo retrieval and multi-class logo detection and object recognition. However, the annotations for object detection were often incomplete,since only the most prominent logo instances were labelled. FlickrLogos-47 uses the same image corpus as FlickrLogos-32 but has been re-annotated specifically for the task of object detection and recognition. New classes were introduced (i.e. the company logo and text are now treated as separate classes where applicable) and missing object instances have been annotated. Furthermore, we provide corresponsing evaluation scripts.
FlickrLogos-32
Description:
The dataset FlickrLogos-32 contains photos showing brand logos and is meant for the evaluation of logo retrieval and multi-class logo detection/recognition systems on real-world images. We collected logos of 32 different logo brands by downloading them from Flickr. All logos have an approximately planar surface.
Classes:
There are 32 logo classes: Adidas, Aldi, Apple, Becks, BMW, Carlsberg, Chimay, Coca-Cola, Corona, DHL, Erdinger, Esso, Fedex, Ferrari, Ford, Foster's, Google, Guiness, Heineken, HP, Milka, Nvidia, Paulaner, Pepsi, Ritter Sport, Shell, Singha, Starbucks, Stella Artois, Texaco, Tsingtao and UPS.
Partitions / subsets:
The retrieved images were inspected manually to ensure that the specific logo is actually shown. The whole dataset is split into three disjoint subsets P1, P2, and P3, each containing images of all 32 classes. The first partition P1 - the training set - consists of 10 images that were hand-picked such that these consistently show a single logo under various views with as little background clutter as possible. The other two partitions P2 (validation set) and P3 (test set = query set) contain 30 images per class. Unlike P1 these images contain at least one instance of a logo but in several cases multiple instances.
To facilitate the development of high-precision classifiers the evaluation of their sensitivity on non-logo images is important. Therefore both partitions P2, and P3 include another 3000 images downloaded from Flickr with the queries "building", "nature", "people" and "friends". These images are the negative images and complete our dataset. A brief summary of the data subsets is shown in the table below.
Partition | Description | Images | #Images |
---|---|---|---|
P1 (training set) |
Hand-picked images |
10 per class | 320 images |
P2 (validation set) | Images showing at least a single logo under various views | 30 per class | 3960 images |
Non-logo images | 3000 | ||
P3 (test set = query set) | Images showing at least a single logo under various views | 30 per class | 3960 images |
Non-logo images | 3000 | ||
P1, P2 and P3 are disjoint. | 8240 images |
![]() |
![]() |
![]() |
![]() |
![]() |
![]() |
FlickrLogos-47
Description:
The dataset FlickrLogos-47 contains photos showing brand logos and is meant for the evaluation of logo detection and recognition systems on real-world images. It is built from the same images than the FlickrLogos-32 dataset which have been re-annotated to fix mission annotations and to include more classes.
Classes:
There are 47 logo classes: Adidas (Symbol), Adidas (Text), Aldi, Apple, Becks (Symbol), Becks (Text), BMW, Carlsberg (Symbol), Carlsberg (Text), Chimay (Symbol), Chimay (Text), Coca-Cola, Corona (Symbol), Corona (Text), DHL, Erdinger (Symbol), Erdinger (Text), Esso (Symbol), Esso (Text), Fedex, Ferrari, Ford, Foster's (Symbol), Foster's (Text), Google, Guiness (Symbol), Guiness (Text), Heineken, HP, Milka (Symbol), Milka (Text), Nvidia (Symbol), Nvidia (Text), Paulaner (Symbol), Paulaner (Text), Pepsi (Symbol), Pepsi (Text), Ritter Sport, Shell, Singha (Symbol), Singha (Text), Starbucks, Stella Artois (Symbol), Stella Artois (Text), Texaco, Tsingtao (Symbol) Tsingtao (Text) and UPS.
Download
Download
If you wish to download one (or both of the datasets), please send an (informal) email to
request_flickrlogos@informatik.uni-augsburg.de. Please state your name, your institution and why you would like to have access to this dataset (we are curious). We then send you a download link by e-mail.
Note: This dataset consists of images downloaded from Flickr. Use of these images must respect Flickr's terms of use.
Important Notes
There is a similar dataset called FlickrLogos-27. It consists of 27 classes only and while there is some overlap it is largely different from our FlickrLogos-32 dataset. Results for these different datasets are not comparable.
Contact
If you have any questions, corrections or other issues please contact Stephan Brehm. The former maintainers were Christian Eggert and Stefan Romberg.