Who we are
We are the Chair of "Networked Systems and Communication Networks" (NETCOM) and focus, among other things, on the measured and perceived quality of networked applications. These include current Internet applications, for which we often encounter network problems ourselves, such as when a video call at home over Wi-Fi is blurry or TikTok videos don't load while on the go in a 5G mobile network. Embedded systems, for example, in the Internet of Things or in the industrial context, also have high requirements for communication networks, necessitating high availability of the communication network or realtime data transmission.
In our research, we investigate how problems in the network can be detected and how the network can be configured to meet requirements, ensuring that issues are quickly resolved or prevented at all. We deal with the classical aspects of network management as defined in the FCAPS model, i.e., our research includes fault management, configuration management, accounting, performance management and security management of user-centric and industrial communication networks using current technologies such as software-defined networks, network function virtualization, and programmable data planes.
The innovations and synergies of our research lie on the one hand in the fact that we not only use objective performance metrics (Quality of Service, QoS) for fault and performance management, but also take into account the subjective experience of the users (Quality of Experience, QoE). Secondly, we use data-driven approaches from the field of artificial intelligence (AI) and machine learning (ML) and adapt them for the monitoring and management of communication networks in order to create fine-grained models that better reflect the high complexity of the interactions between users, applications and networks than previous models. Despite the increasing encryption of data traffic, technical parameters and performance indicators of the applications can be estimated with a high degree of accuracy in a privacy-preserving manner, which enables application-aware network management.
To achieve our research goals we employ a wide range of methods. We conduct research using model based approaches through theoretical considerations or simulations, data driven methods employing Data Science and AI/ML, and system based approaches involving realistic implementations and measurements in testbeds with actual hardware.
Our vision is that future networked systems and communication networks will be able to configure themselves flexibly, autonomously and proactively by learning from available and monitored network data, so that the performance, reliability and security of networked systems and the QoE and satisfaction of users are optimized.
News
Tutorial on XAI for QoE Modeling
In research, it is important for us to know which factors have the greatest impact on user satisfaction and what fundamental relationships are hidden within the data. This is where methods from explainable machine learning (XAI) can help, and we have written a tutorial paper on how such methods can be applied to QoE modeling.
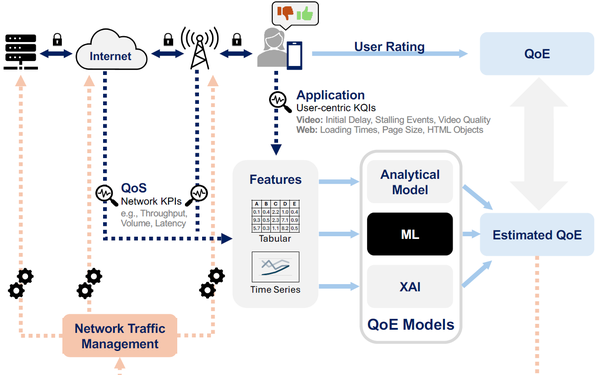
NETCOM summer party 2025
On Friday, July 4, it was time again for our second NETCOM summer party! The focus was on fun and getting together. We started at the Wittelsbacher Park with games outside in the beautiful weather, before ending the day with a nice meal and relaxed chats at the Old University.
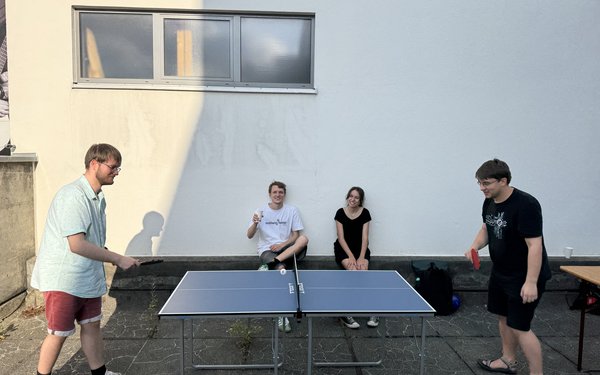
SLICES PhD Summer School
From June 25 to 27, 2025, Filip participated on the SLICES-RI / CONVERGE PhD Summer School in Porto, Portugal. The event was hosted by the research institute INESC TEC, located on the campus of the Faculty of Engineering at the University of Porto.
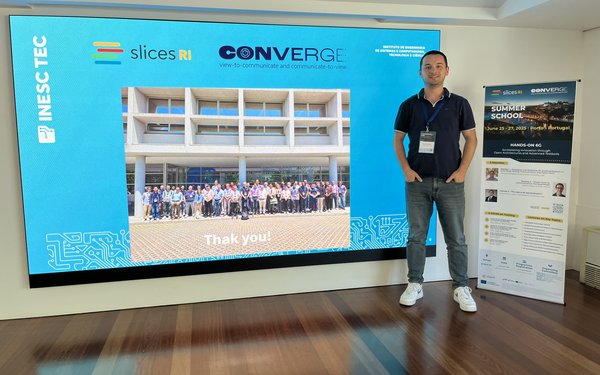
Contact
- Phone: +49 821 598-2793
- Email: michael.seufert@uni-auni-a.de ()
- Room F2-403 (Building Standort "Alte Universität")
How to reach us:
Postal address (post office box):
Universität Augsburg
Institut für Informatik
Lehrstuhl für Vernetzte Systeme und Kommunikationsnetze
86135 Augsburg
Building address:
Universität Augsburg
Institut für Informatik
Lehrstuhl für Vernetzte Systeme und Kommunikationsnetze
Eichleitnerstraße 30
86159 Augsburg
Building: F2 - 4th floor
Phone: +49 821 598 - 5541 (secretary)
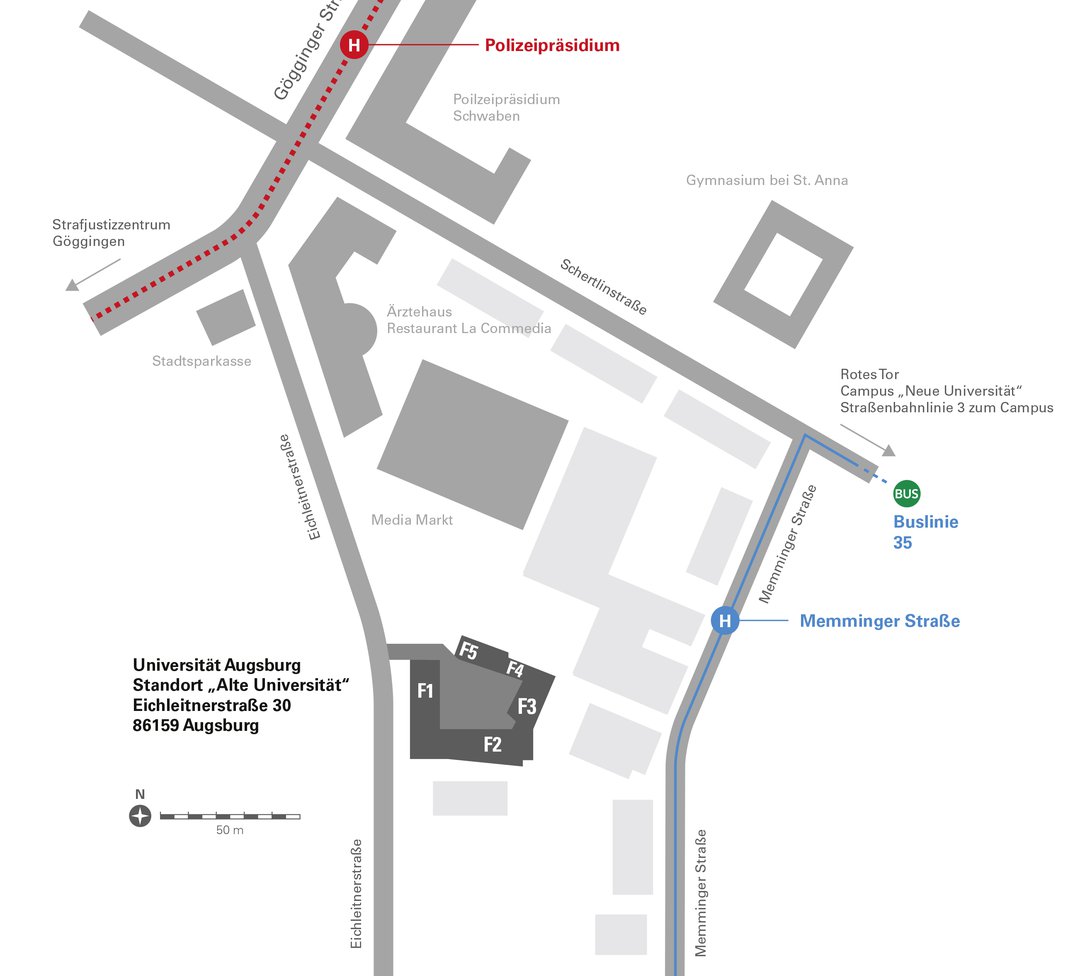